Introduction to machine learning in commercial real estate Part 1: Features, competitive advantages and range of uses
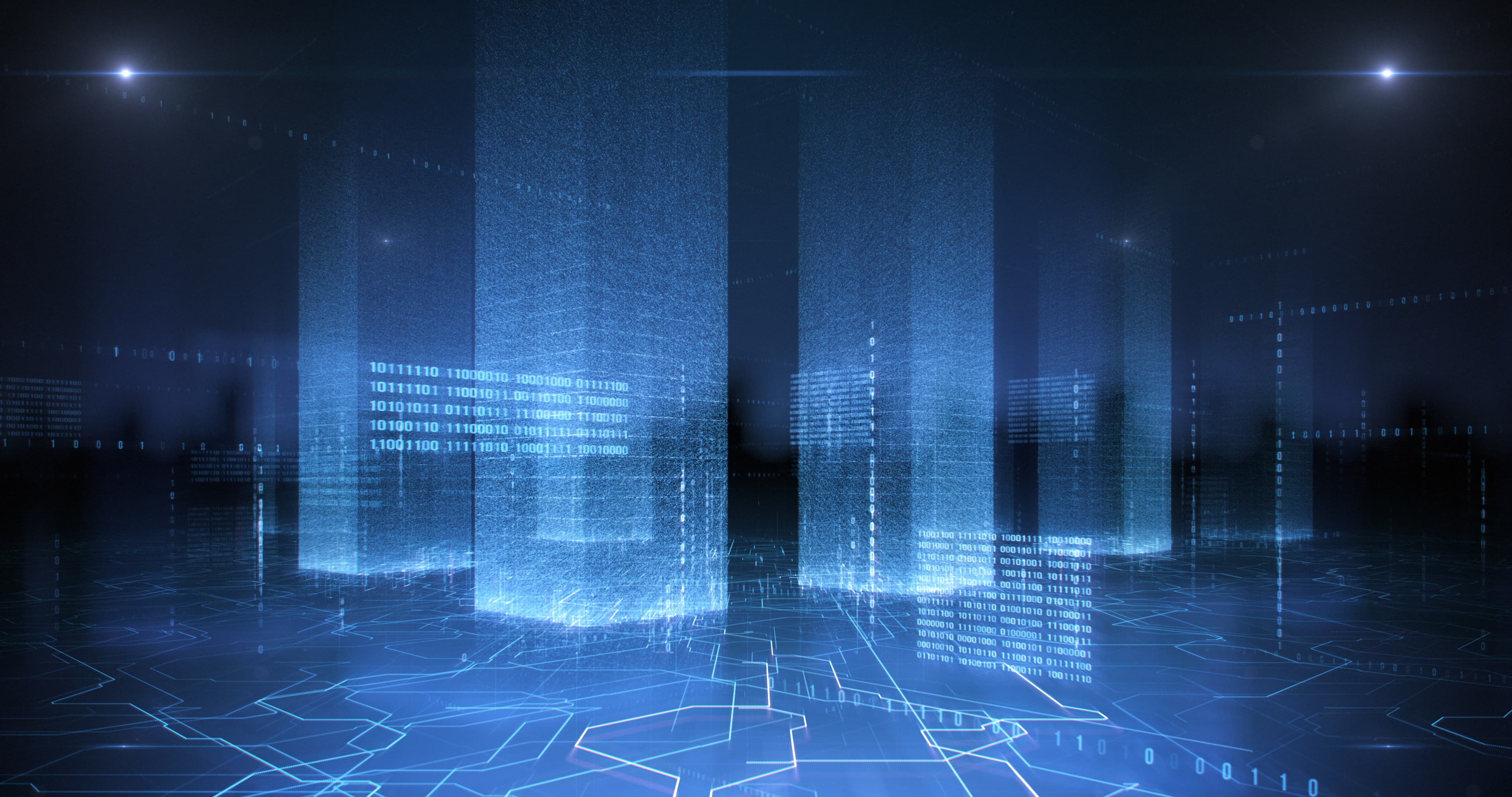
Key highlights
Machine learning (ML) is becoming essential in CRE decision-making, providing faster and deeper insights into investments and creating a widening gap between early adopters and those who lag in implementing ML strategies
ML helps CRE businesses clarify performance, identify trends, evaluate investments, estimate value and risk, and improve property management
Despite the benefits, CRE firms have been slow to adopt machine learning due to challenges such as a lack of quality data, organizational buy-in, and difficulty in hiring qualified staff
Multi-faceted changes in the market are accelerating the need for enhancements in investment analysis
The commercial real estate (CRE) industry is at the epicenter of change and stress.
Accelerating globalization
Demographic and geopolitical shifts
Rising cost of capital
Expanding risks
Talent shortages
Digital transformation
Evolving demand for space
Hyper-competition for deals
Escalating pressure on returns
As these dynamics drive change in the industry, CRE owners, investors, analysts, and managers need to make nimble, discerning decisions. The vast volume of commercial real estate data accessible for investment analysis underscores the necessity for automated research procedures. These processes reduce the efforts spent sorting through data, thereby allowing more time for crucial decision-making concerning asset allocations, acquisitions, dispositions, and overall asset performance.
In what ways is that market changing? Should we sell that building? When? Or will net operating income increase next year? What’s influencing the lower performance of those properties? Should we move out of the office class and into multi-family? When would be the optimal timing for disposition?
You want to be confident that your investment strategy is responsive to current market needs and challenges, and is supported by the best data available. So, in the face of continuing evolution and pressure, how can real estate businesses quickly find hidden patterns—and harness them to make these decisions for profitable investment?
Machine learning is one of the key tools. Machine learning sifts through vast amounts of complex data to uncover patterns, correlations, and other insights that facilitate immediate, accurate assessments and predictions – a critical advantage in a volatile CRE environment. This can accelerate research and analysis, reduce risk, and improve efficiency in property valuation, management, and investment.
Machine learning terms and definitions
Artificial intelligence is a set of technologies that enable a machine or system to learn, reason, and solve problems.
Machine learning is a component of artificial intelligence that enables a machine or system to automatically learn and improve from experience, rather than being programmed by people.
Machine learning is also sometimes referred to as predictive analytics or predictive modelling.
Machine learning uses algorithms (instructions for solving problems) to analyze large amounts of data, learn from this, and then make informed decisions. As they are exposed to more data (trained), through a process of trial and error, algorithms improve performance and produce increasingly accurate results.
Training data is an initial set of data samples used to train machine learning algorithms by example. Using this data, machine learning models develop and refine rules.
Test data is an independent subset of the training dataset which is utilized as an objective evaluation to verify the accuracy of the final model.
Machine learning models are the output – what the program learns from running an algorithm on training data. High-quality data of a sufficient sample size is critical for creating accurate, useful models.
Supervised machine learning uses labeled datasets (descriptive labels added to raw data for context) to train algorithms to classify data or predict outcomes. With labeled inputs and outputs, the model can calculate accuracy and learn over time. This is the type of learning most commonly used in machine learning.
Unsupervised machine learning analyzes unlabeled datasets (pieces of raw data that are not tagged with identifying characteristics), organizing them based on similarities and differences.
The target variable is the feature of a dataset you want to understand – the variable the model is trying to predict.
Given the potential benefits of machine learning for CRE, uptake should be higher
While it is the largest investment asset class in the world, real estate trails most industries in deploying data and advanced analytics to support strategic decision-making.
Meanwhile, the sources and uses of data in the real estate world are flourishing. Along with traditional household, demographic and economic data, new data sources are increasingly available for identifying granular information that impacts properties – down to the level of a city block. This includes data related to cellphone use, foot traffic, accessibility to points of interest, power consumption, broadband subscriptions, subway and train volumes, even credit card transactions.
“Machine learning thrives on vast amounts of data. The more high-quality data it has, the more accurate the results."
Machine learning also enables the analysis of new forms of data. For example, until recently, information formats such as images couldn’t be used as data inputs. But now, machine learning algorithms can identify elements within images such as the number of vehicles in a parking lot. Until recently, unstructured data could not be used as data inputs. But now, Optical Character Recognition (OCR) can be utilized to extract text from unstructured data, such as tax bills or property records.
As well, machine learning models can forecast key factors, such as household net worth, significantly more accurately – as much as 20 times – than traditional models.
Within the ever-growing mountains of disconnected data from disparate sources, making sense of what’s relevant and irrelevant is overwhelming – without the assistance of machine learning. To discern clear patterns and glean insights requires sifting through tens of millions of data points. By the time many CRE businesses collect, compile, and process the data needed to do this, the best opportunities are gone.
Altus’ 2021 CRE Innovation Report found that 25% of CRE senior executives around the globe were using machine learning in a significant way, 22% were trying it out and 37% were considering starting to use it within 18 months.
Given the potential benefits of machine learning, these numbers should be higher. Some hesitation may be due to a need for a greater understanding of use cases for this technology, which demonstrates how it can open new opportunities that deliver significant value and competitive advantage.
Toward this clarity, the following are examples of how machine learning can support timely, intelligent real estate investment management.
How CRE firms can use machine learning to advance their competitive advantage in the current economic climate
By analyzing varied and massive volumes of disparate data, machine learning can perform a range of forecasting and financial modelling processes to support investment acquisitions and dispositions and to optimize operations.
Identify outperforming/underperforming assets: Machine learning yields insights into hidden market factors that influence portfolio and asset performance. For example, by examining criteria such as rental income, occupancy rates, net operating income, and maintenance costs, ML can project property returns and identify which assets are performing above or below average, enabling timely decisions to hold, sell, or improve properties. While traditionally these types of financial models were generic, we can now use machine learning models for individual projects or buildings. This yields a precise picture of its associated rewards and risks. Such detailed financial modeling can even help to secure optimal project financing.
Identify market trends and evaluate potential: Machine learning can predict market dynamics and trends such as changes in demand, property value, rent price fluctuations, and leasing activity, intended to identify optimal timing to buy or sell properties. It can compare markets and neighborhoods based on growth and risk scores and performance forecasts. It can also be used to forecast market downturns, property appreciation or devaluation, even tenant defaults.
Determine optimal asset classes for investments: Using market trends, demographic and other data to identify patterns, machine learning can assess growth or demand for specific asset classes or subclasses in particular markets. This can be invaluable for predicting demand for specific property types and for the timing of acquisitions, dispositions, or reallocation of assets.
Prospect properties: Machine learning algorithms can process a range of near- or real-time economic, demographic and property data at granular levels of geography to identify locations with the highest demand for specified types of assets. And it can improve deal sourcing by analyzing datasets across many markets. Machine learning can also facilitate rigorous due diligence by comparing current and potential property performance based on a large range of factors. In the case of multi-family housing, for example, this could provide a view of the specific number of people currently moving into a particular city or region, the type of housing they are moving into, where they are from, their median age, household income, net worth, home value, employment sector, even their level of education – and enable you to identify buildings in areas that are currently undervalued but rising in demand.
Estimate property value and risk: Harnessing historical sales data and a range of criteria impacting property price, including demographics, location, size, amenities, economic indicators, recent sales information, and other related factors, machine learning can produce precise, human-bias-free property valuations. Machine learning can also rank search results, categorize properties, and indicate comparable properties. And it can assess economic indicators to identify risks associated with investing in a particular market or property.
Increase deal volume: Using customized search criteria, machine learning can help to identify large pools of potential investment targets, and then rapidly shortlist them.
Determine rental rates: Algorithms can combine rental information from sources such as market trends, real estate listings, property type, and lease contracts to closely estimate rental rates.
Improve property management operations: By leveraging data related to occupancy, rental rates, and tenant behavior such as rent payment activity, lease length, and satisfaction surveys, machine learning can improve the efficiency of property management operations. This can range from lease renewal and rent collection to maintenance scheduling and financial reporting.
Machine learning models clarify real estate performance and projections
Traditionally, growth projections in the commercial real estate sector were based on linear regression – the relationship between two variables. The price of property A, for example, increased 2% annually over the past five years so based on linear regression it can be expected that it will increase by 2% next year. A single input variable is used to predict the output variable.
But if you want to expand a portfolio of multi-family buildings, drawing clear hypotheses and building a robust business case would be challenging with such conventional data and analytical methods.
Now, machine learning predicts output based on multiple inputs and reflecting more complex interactions so you can assess the influence of many factors, from population growth to property features, to interest rates, along with the degree to which each of these factors relates to the others and affects overall growth. This produces a significantly more accurate picture than simple linear regression.
Machine learning enables advantageous decisions
As the quantity and quality of data continues to progress and the real estate environment becomes more complex and pressurized, machine learning will play an increasingly critical role in enabling CRE businesses to acquire faster, deeper insights about investments. This will widen the gap between early ML adopters and laggards.
Challenges create opportunities to change and grow – to think through new strategies, to test new theories.
In today’s capricious real estate environment, examining the potential of machine learning to enable advantageous decisions that add value to your real estate assets isn’t simply innovative, it’s astute.
Read part 2: Five components to leverage machine learning advantages
Author
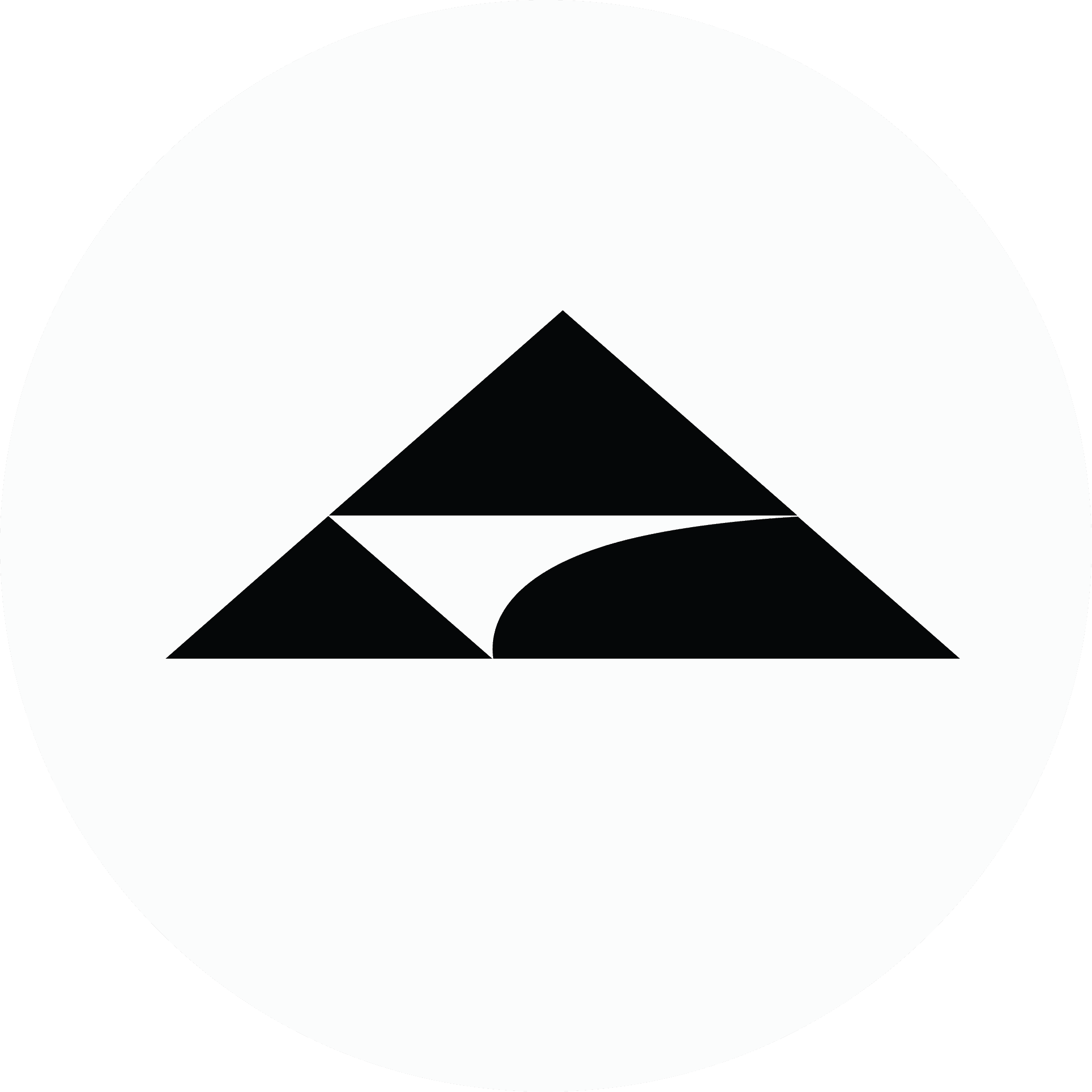
Altus Group
Author
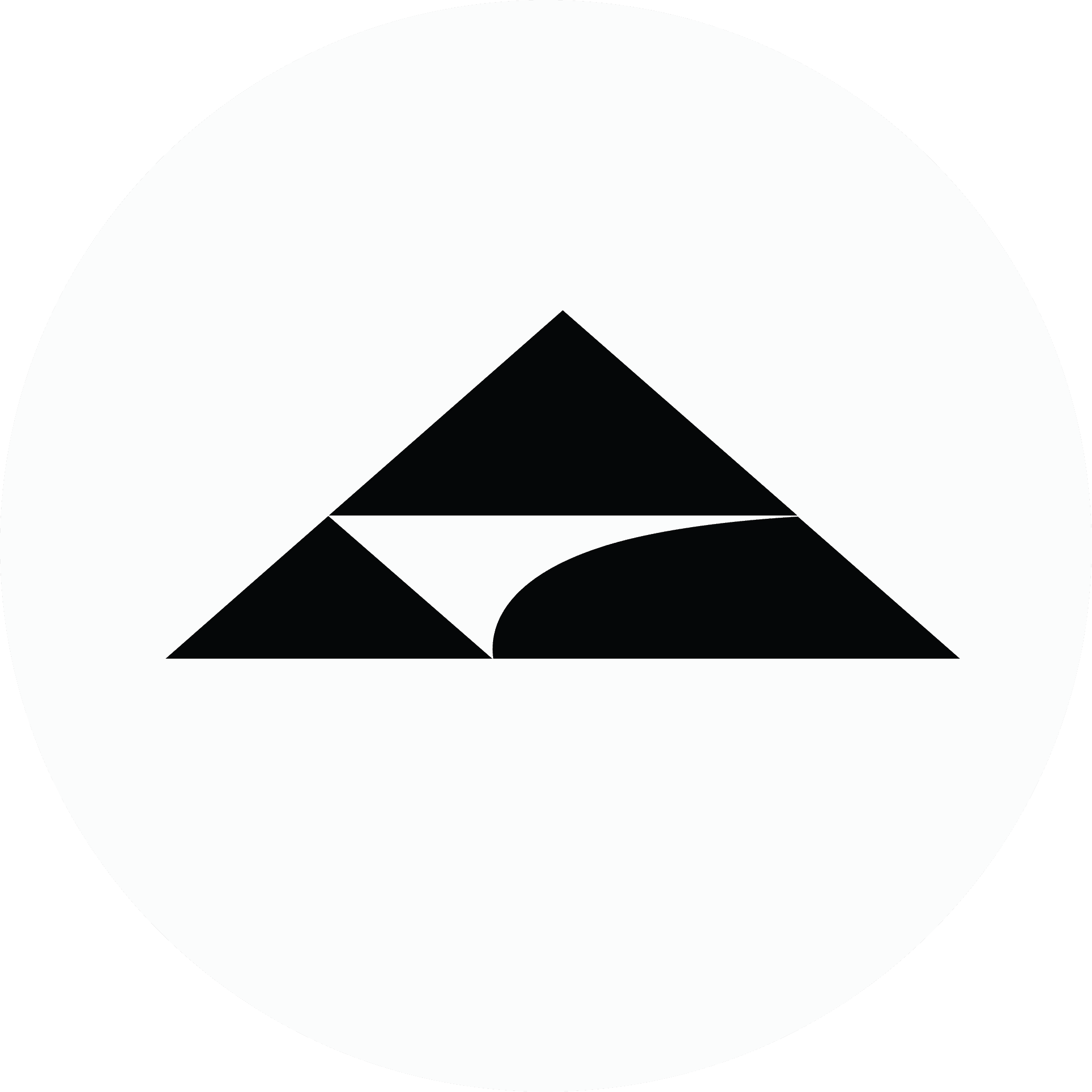