A North American perspective on data science in CRE
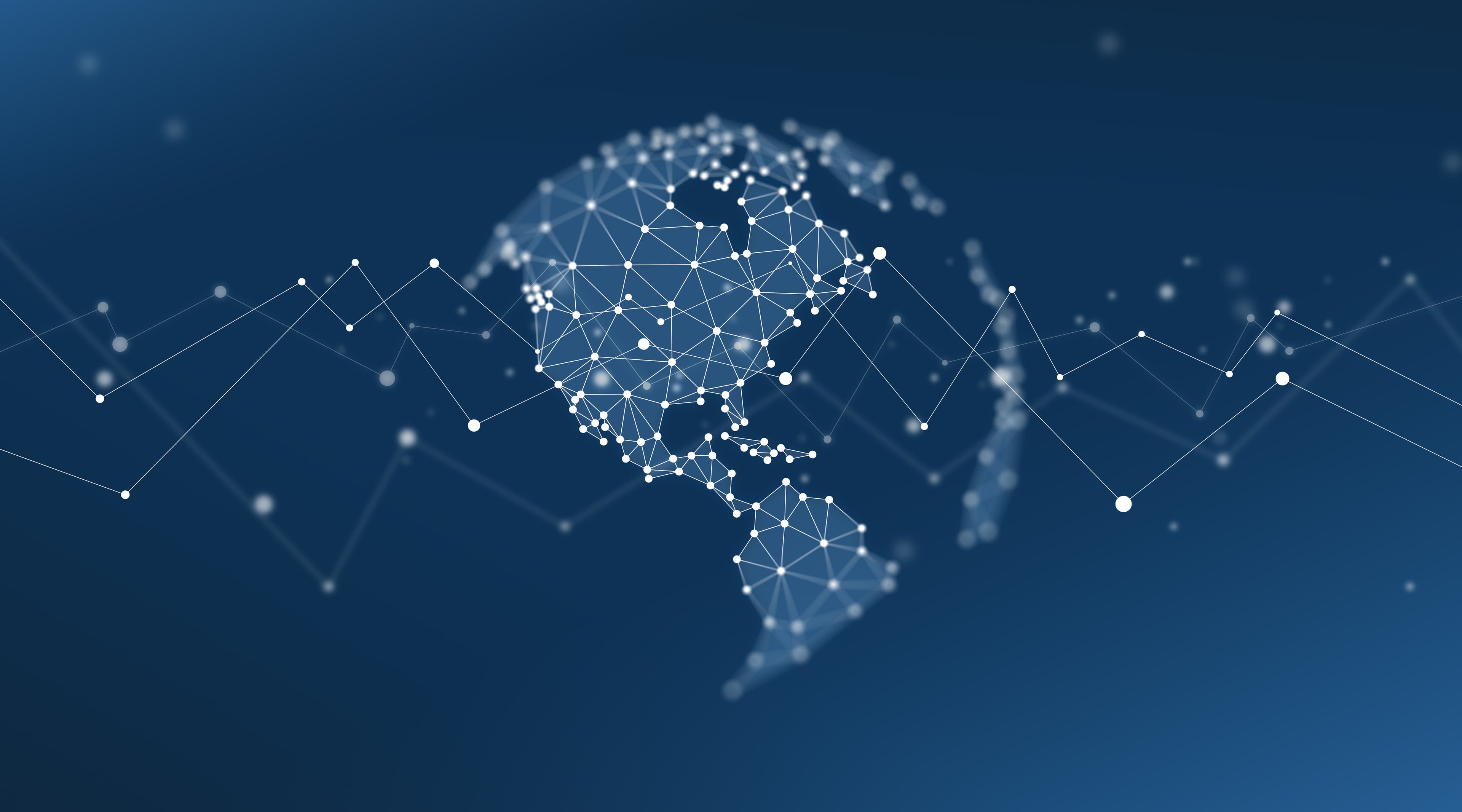
Altus asked me to look over their recent research report on data science in commercial real estate and give my take on the results. I’ve been working at the intersection of real estate and technology for about six years and my experience is pretty much in line with the results of the surveys contained in the report – namely, a lot of confusion and uncertainty about what’s going on and what to do next.
While the report covers quite a few areas regarding how firms feel about how well they’re currently implementing technology and what their plans are for the future, I want to discuss a few that I see daily and that I think are major impediments to any real transformation of the real estate industry through the use of data and technology, including:
Unrealistic expectations about what technology can do and what it takes to develop it effectively
Big misconceptions about where firms are in the technology development and adoption process
Disappointment in the current state of the use of technology in real estate
Lack of clarity about what to invest in
I’ll cover each of these in more detail below, but I think there are two core reasons that explain why real estate firms are having such a difficult time with the development and integration of technology. The first is the lack of technical knowledge regarding data, data science, and data analytics methodologies within real estate firms and the industry in general. The second is that real estate involves a type of complexity that is vastly different from other industries that have been successful in their use of technology. This means that using approaches that have been successful in other industries don’t always transfer well to real estate.
And because much of the real estate industry is unfamiliar with how to work with data in the context of data science applications, how to build pipelines conducive to effective analytics, and how to build learning algorithms that can be converted to working models for real estate, firms often misunderstand the technical approach that’s needed to solve complex real estate problems through the application of data science in the industry. Keep these in mind as we discuss each of the items above.
Unrealistic expectations lead to constant disappointment
Real estate firms must be very clear about what problem they’re trying to solve, what data or functionalities are needed to solve that problem, and what data/service providers offer the tools to address the problem effectively. Developing an understanding of what data is needed to solve the problem requires an understanding of both the real estate problem and the technical approach, which is something that real estate companies, again, usually do not have in-house. Some functions can be solved with the right data/service provider, but not all data/service providers are right for all problems.
The other misconception is about the time, skills, and resources necessary to develop strong analytical capabilities. Building good technical capabilities requires years of refining a pipeline consisting of problem structuring, data collection, data cleaning, optimizing the storage of data, tweaking and iterating learning algorithms until they’re acceptable, and then converting those learning algorithms and data to models that can be used in the real world. This process is not optional. It’s necessary to develop working analytics capabilities and it’s not easy, cheap, or quick, but there’s no shortcut.
One section of the report summarizes the challenges that respondents were having when attempting to develop data science capabilities internally.
Figure 1 - Challenges and pain points with internal capabilities
The ironic thing about this part of the report is that respondents to the survey cited most of the reasons that developing analytical capabilities are so difficult. First, real estate lacks high-quality data (mentioned by 44% of respondents). Second, most firms don’t allocate anywhere near the level of resources needed to successfully complete analytics projects. Building successful analytics capabilities takes time, money, and a group of people with the right skillsets. A data science intern for the summer is not going to work. The lack of organizational buy-in and support was mentioned by 41% of respondents.
One reason for the mismatch between expectations and resources has to do with the “transformation” and “disruption” that the “proptech” world has promised. According to many in the industry, however, proptech hasn’t yet delivered anything close to what would be called transformative capabilities. Some processes have become more efficient and there has been some improvement, but it’s been minor relative to the claims and expectations about what benefits technology would provide the industry. These unrealistic claims and expectations are not harmless. Because they are unrealistic, firms tend to believe that the investment in terms of people, money, and resources needed to successfully pursue data analytics projects are significantly less than what is actually needed. This under-investment in resources often leads to unsuccessful technology development initiatives that then lead to disappointment within firms.
So even though there’s a recognition of the challenges associated with building internal data and technology capabilities, it seems most firms still aren’t allocating the resources needed to do it successfully.
Confusion about current capabilities
According to the survey, 73% of real estate firms think that they’re ahead of the curve in the use of data science. I think these responses are understandable if they’re based on a relative view compared to other real estate firms, but certainly inaccurate if you consider where firms are compared to where they need to be to foster, develop, and integrate effective technology.
Figure 2 - Organization's approach and attitude towards data science/analytics tools for real estate investments
What’s more interesting is that even though 73% think they’re ahead of the curve in applying data science, only 55% of respondents selected one of the options that Altus considers a “data science capability.” So if 45% of respondents are not using data science capabilities, but 73% are ahead of the curve, what are those firms referring to when claiming to be more advanced than their peers? I think there’s an easy explanation for this, though. Because most real estate firms lack deep technical expertise as I mentioned above, they don’t recognize that the processes they currently have in place are still drastically insufficient for robust analytics capabilities.
Figure 3 - Data science and analytics capabilities organizations currently employ to support real estate decision-making
What real estate firms must do to better prepare for digital transformation
Over the past ten years or so of the technology movement in real estate, most firms have overwhelmingly looked to external data and service providers as the source of their “becoming digital.” And most firms have expressed immense frustration at the impact much of this external technology has had on their firm and the industry and I think this is due to three reasons.
First, firms don’t have strong internal technical infrastructures suited to data analytics, which makes it difficult and much more costly to integrate new technologies successfully (discussed more below). Second, there is a ton of noise from “startups” offering products and services that the industry has not found valuable. Firms need to sift through providers for those with relevant products and services rather than choosing those that tell good stories. Third is the gap mentioned above between expectations and reality.
While many of the firms surveyed said they plan to invest in some mix of both internal and external analytics capabilities in the future, these plans need to be structured with much more clarity than I’ve seen in most real estate firms. The reason is that the integration of both internally and externally developed technology is extremely difficult for firms that don’t have robust internal technology capabilities. Who’s going to tie together functions in different parts of the firm? Who’s going to integrate new systems with old systems? While most real estate firms think this will easily and quickly be done by external service providers, this is rarely the case.
In my opinion, real estate firms first need to develop robust internal technology capabilities. The first step to that is fostering a more technical culture, meaning education for employees and executives alike. Employees who can recognize viable use cases for technology can greatly facilitate the development and use of new capabilities. Executives who can think strategically about how to use technology to give their firms new opportunities rather than just replace old systems with new systems that accomplish the same thing will be able to provide competitive advantages to their firm.
Conclusion
I found this report very interesting because it quantitatively supports what I’ve personally experienced and what I’ve heard anecdotally from many people in many areas of the real estate and proptech industries. A thorough discussion about what the proptech movement is doing right and what it’s doing wrong, as well as ways the approach can be improved, could take up hundreds of pages. What I’ve discussed in this article are the ones that I feel most need to be addressed in order to improve the efficacy of data science activities within the industry. The report can be found here and I recommend checking it out for a lot more detail on how firms are feeling about data science in real estate.
About the author
Josh is currently a member of the Center for Artificial Intelligence in Business Analytics and Financial Technology where he serves as the Director of Real Estate Artificial Intelligence Research & Innovation in the School of Engineering and Applied Science (“SEAS”) at Columbia University. He joined Columbia in 2016 and is the former Director of Real Estate Technology Initiatives at SEAS. His focus is on using practical applications of artificial intelligence and machine learning to address inefficiencies and create new capabilities within real estate.
Prior to academia, Josh spent 12 years in various roles in real estate. He was Head of Credit Modeling and Analytics at Deutsche Bank’s secondary CMBS trading desk, where he helped develop and implement automated models for CMBS loan and bond valuation. He also worked at the Ackman-Ziff Real Estate Group and in various other roles in research, acquisitions, and redevelopment. Josh has a master’s degree in finance from San Diego State University, a master’s degree in real estate finance from New York University’s Schack Institute of Real Estate, and is currently pursuing degrees in applied mathematics and data science at Columbia University.
Author
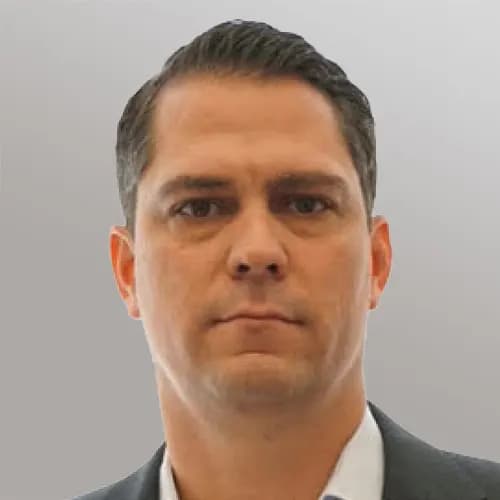
Josh Panknin
Director, Real Estate AI Research & Innovation at Columbia University Engineering
Author
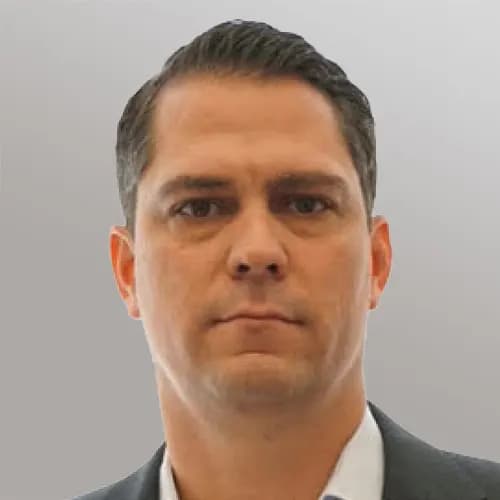
Josh Panknin
Director, Real Estate AI Research & Innovation at Columbia University Engineering
Resources
Latest insights
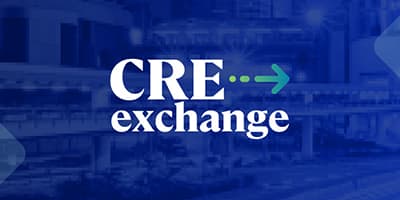
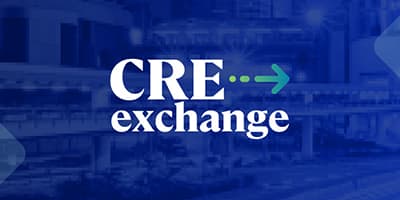
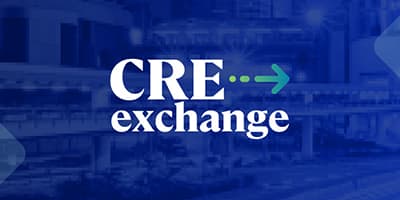
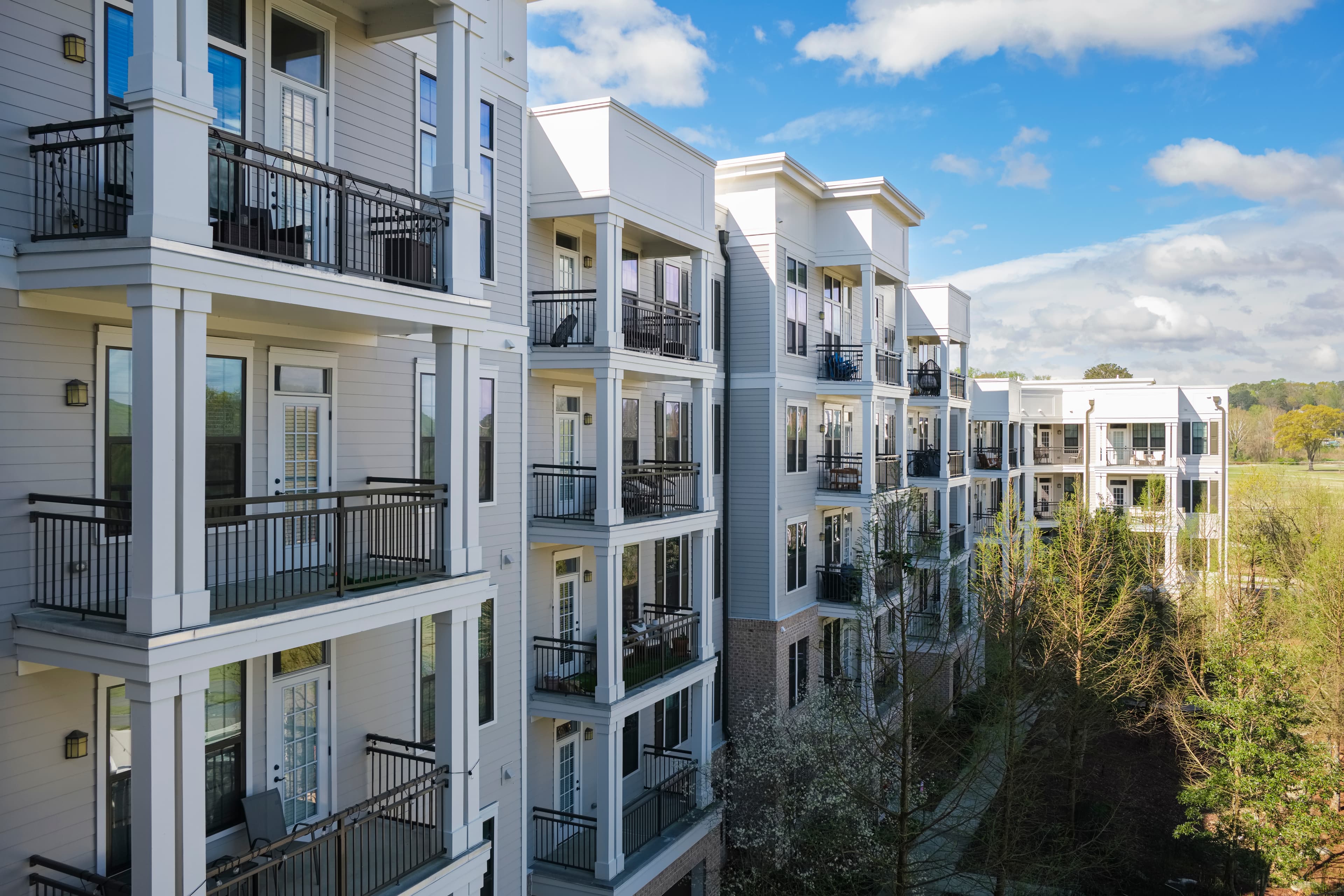
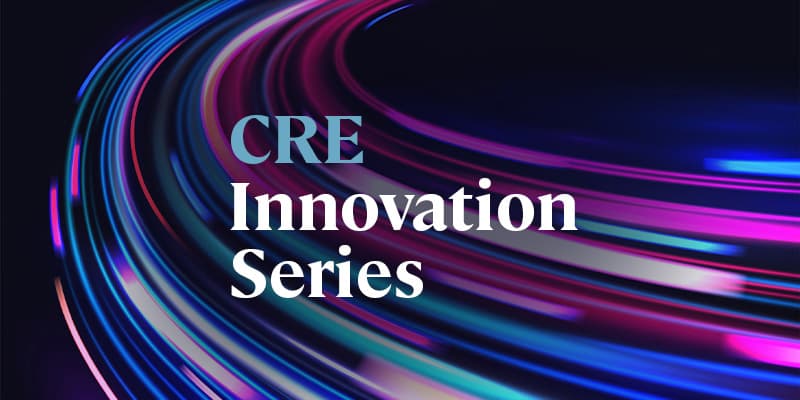
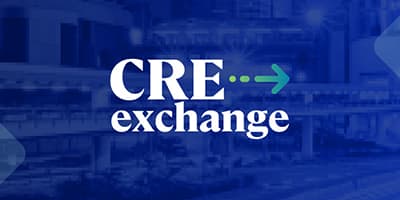