Managing data sources effectively is crucial for building the foundation for analysis that drives strategic and operational decision-making.
Real estate organizations that can interweave different data from different places are able to produce more accurate analyses and generate efficiencies, consistent reporting, and greater insights for decisions.
To successfully drive a strategy for data and analytics excellence, we’ve identified five fundamental elements critical to overcoming barriers and progressing along your transformation journey.
This insight explores the second fundamental: managing your data sources.
Seven steps to managing data sources
Source the right data by starting with the desired outcome
Profile the data you need
Leverage reliable third-party date
Establish quality controls
Eliminate data silos with centralized repositories
Embrace technology for repetitive tasks
Normalize databases
Why is managing data sources important?
Appropriate, high-quality data are critical for realizing potential. Processing irrelevant, invalid, incomplete or incorrect data can lead to faulty analyses – and poor outcomes.
Yet consolidating data from disparate sources can be challenging, especially as the number of data sources is growing exponentially: internal and external databases, web applications, CRM systems, and more. And extracting, transforming, and making data compatible with your systems can be time and resource-consuming.
"Repeatable data is important for forecasting. You need to be able to get data from one source on a regular basis so you don't have to rewrite templates and reports every time"
1. Source the right data by starting with the desired outcome
Start data sourcing by asking what business outcome you want to achieve. Then determine what data you need to support this outcome. Where can you source this data?
A typical real estate fund might need a range of data that includes:
Market intelligence for benchmarking
Demographic information for projections
Economic data, such as the consumer price index and inflation rate that are required to prepare forecast or valuation assumptions
Debt information from the treasury department
Budget information from the accounting department
Internal and external property valuations from analysts
Building operations information such as expenses and lease information, from property managers
Tax information from the tax department
Audited financial statements from the auditors
2. Profile the data you need
When ingesting data, it is important to ensure that it will meet your requirements to avoid creating a “data swamp.” This refers to dumping data into databases, data warehouses, or data lakes without a clear business need, and subsequently having to perform time-consuming sorting and cleaning processes.
Instead, profile the data you require: its frequency, structure, format, granularity, and method of delivery. When acquiring data from external sources, ensure the provider understands your goals for the data and can meet these requirements.
3. Leverage reliable third-party data
When you need to supplement your own data sources to fulfill your strategies, a third-party data provider can help to meet these needs. Moreover, it can be expensive to establish your own collection system for external data such as market intelligence, demographic and economic data.
Third-party firms can supply subscriptions with accurate, up-to-date data, which includes aggregating and cleaning the data for their clients. Additionally, they may offer expertise related to effectively managing these data sources.
Here are some factors when choosing a provider:
Quality: Where they source their data, how it is collected, and what methodologies they apply; for example, if you need demographic data, recency, granularity and accuracy are critical
Format: Compatibility of data formats can facilitate a smooth transition from external provider to internal use
Frequency and repeatability: To ensure you can acquire the necessary data for your timing needs
Scale: The amount of data they have available as your data use expands
Reputation: Who they work with and the results achieved in those engagements
4. Establish quality controls
Address data quality issues as soon as possible in the data supply chain, before they become problems, by establishing quality controls. For example, you can establish metrics for data quality and implement processes to verify source data against these metrics.
5. Eliminate data silos with centralized repositories
Internal data silos often cause problems for data analysis because valuable data is commonly spread across multiple physical locations and different repository types. Moreover, information is typically stored in formats that are incompatible with one another.
This creates both data sprawl and fragmentation. Users may not know where to find the right data and when they do find it, may have to spend substantial time gathering from multiple sources.
Data warehouses and data lakes are becoming more common for this reason. Both are centralized repositories for storing enterprise data from a broad range of operational sources. As well, both can integrate algorithms to drive real-time analytics.
Figure 1 - Data warehouse and Data lakes
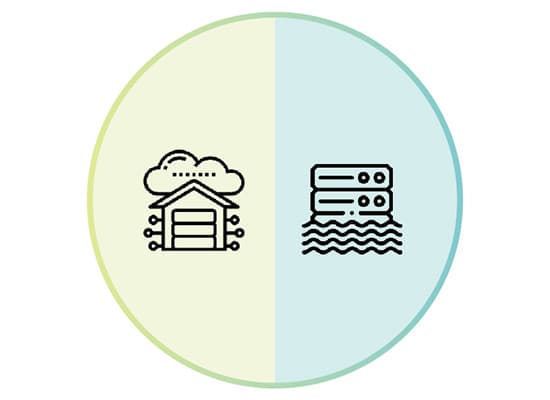
Data warehouses maintain structured, filtered data that has already been processed or formatted for specific purposes such as financial and regulatory reporting.
Data lakes are pools of raw, granular data, whose purposes have not yet been defined. These are growing in popularity data is stored in its pure form, enabling users to spend less time restructuring data.
Typically housed in cloud-based, distributed storage, they make data available to any authorized stakeholder. This also encourages internal collaboration and facilitates productivity because multiple users can share data on the same system.
6. Embrace technology for repetitive tasks
Manual, repetitive tasks such as data migration and cleaning are inefficient and prone to error. Data cleaning is often needed to ensure data are correct, consistent and usable and to reduce the margin for errors in data analysis. Unfortunately, for many organizations cleaning data, especially for large data sets, takes up more time than analyzing data, wasting valuable time and resources.
Intelligent tools can provide end-to-end data-cleaning solutions that identify and rectify inconsistencies. This clean data can then be converted into a suitable format and loaded into the database, warehouse or data lake.
"Enter it once and use it many times. Ideally, you want data to be able to be shared with every system in your environment from asset management to accounting. Each group should be able to look at that data from their own lens and apply what is important to them"
7. Normalize databases
In many situations, internal databases are outdated or poorly designed, which can cause repeated problems while adding, deleting or updating information. These also create challenges for the individuals who utilize these databases for queries and analysis. Normalizing databases eliminates redundancies, resolves conflicting data and also formats the data to expedite processing and analysis.
"Marrying and normalizing data can be a lot of work. When you're dealing with data from many sources, you can have a huge clean-up task. Many analysts spend the majority of their time just prepping data."
Next steps
Managing your data sources can be challenging, and time and resource consuming. But once you can manage high-quality data, the benefits will pave the way for realizing the full potential of real estate organizations. Analysis will become more accurate, processes will become more efficient, and insights will lead to more informed decisions. By implementing the six steps outlined above, you can start to manage your data sources effectively and capably.
Now that you have started managing your data sources, take the next step in your data transformation journey and learn about the third fundamental: How to establish a data governance framework for your CRE business
Author
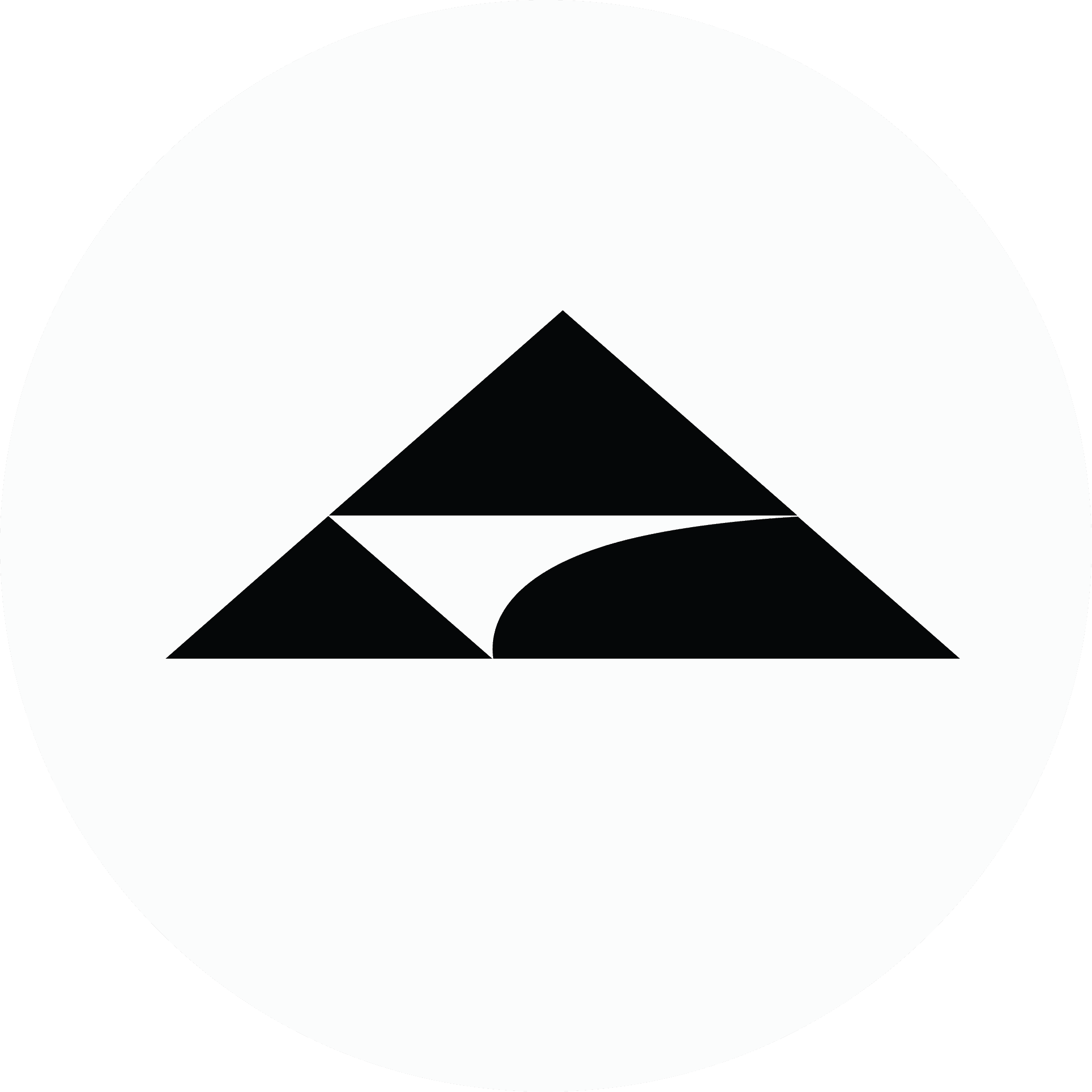
Altus Group
Author
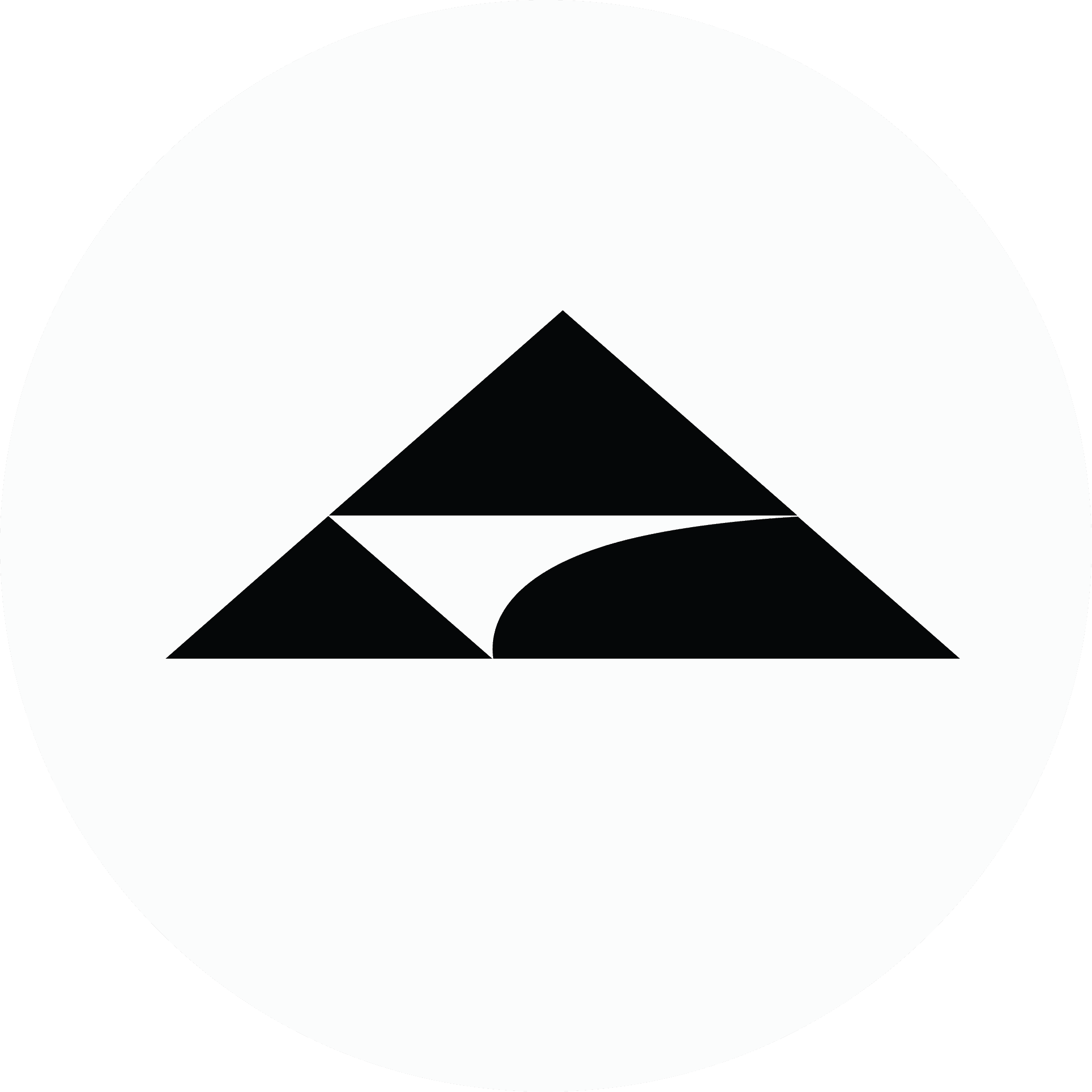
Altus Group
Resources
Latest insights
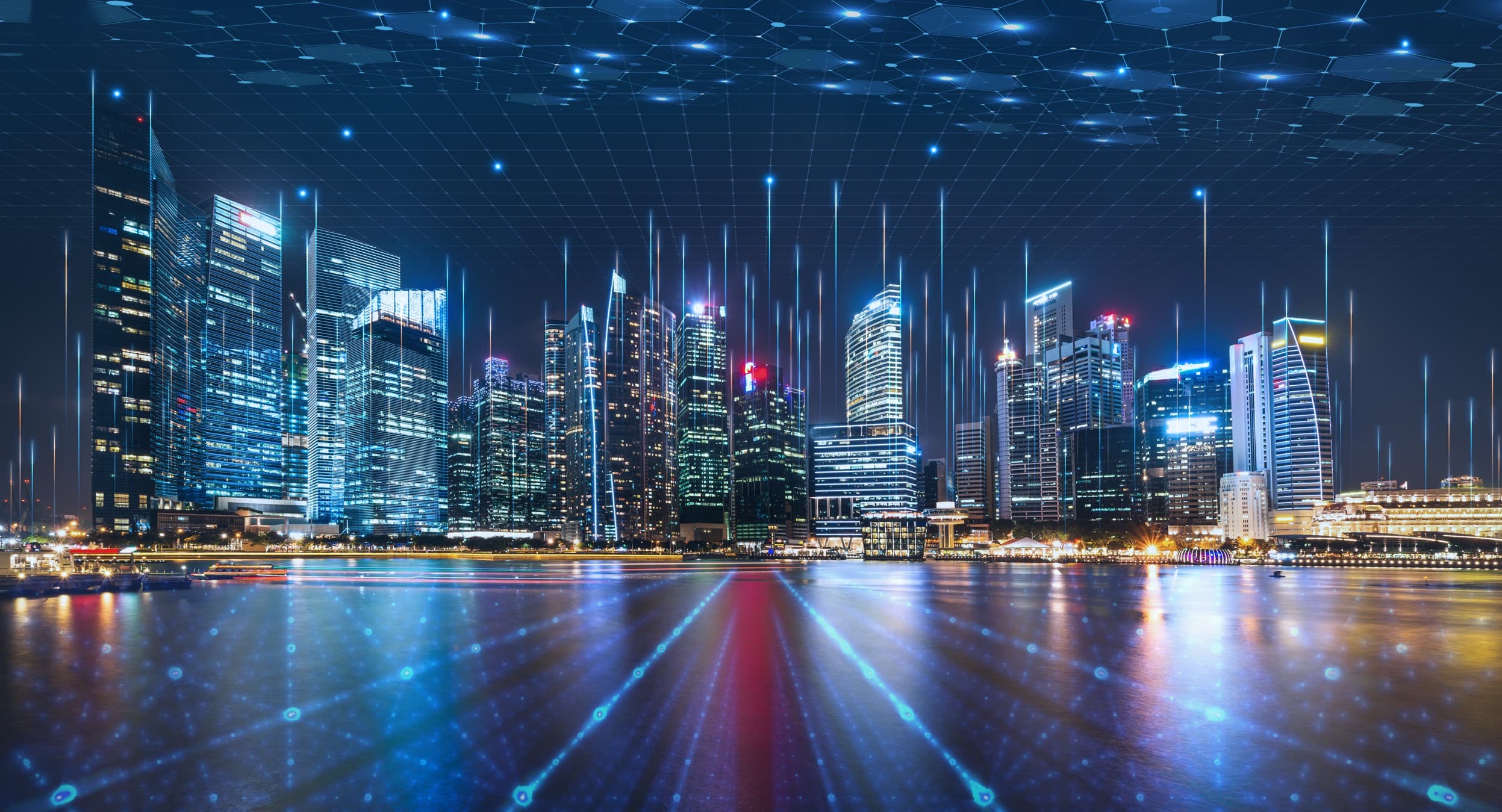
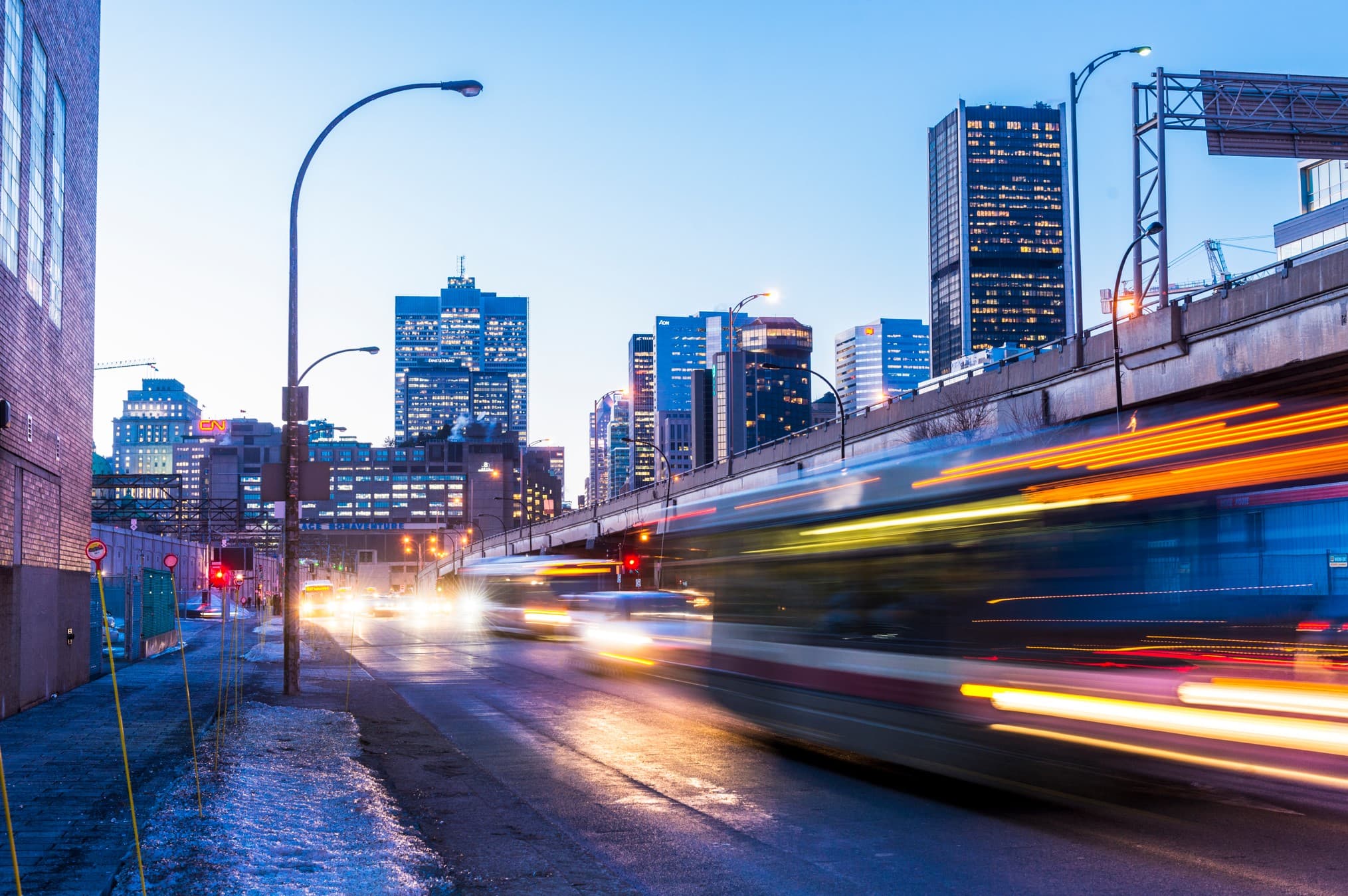
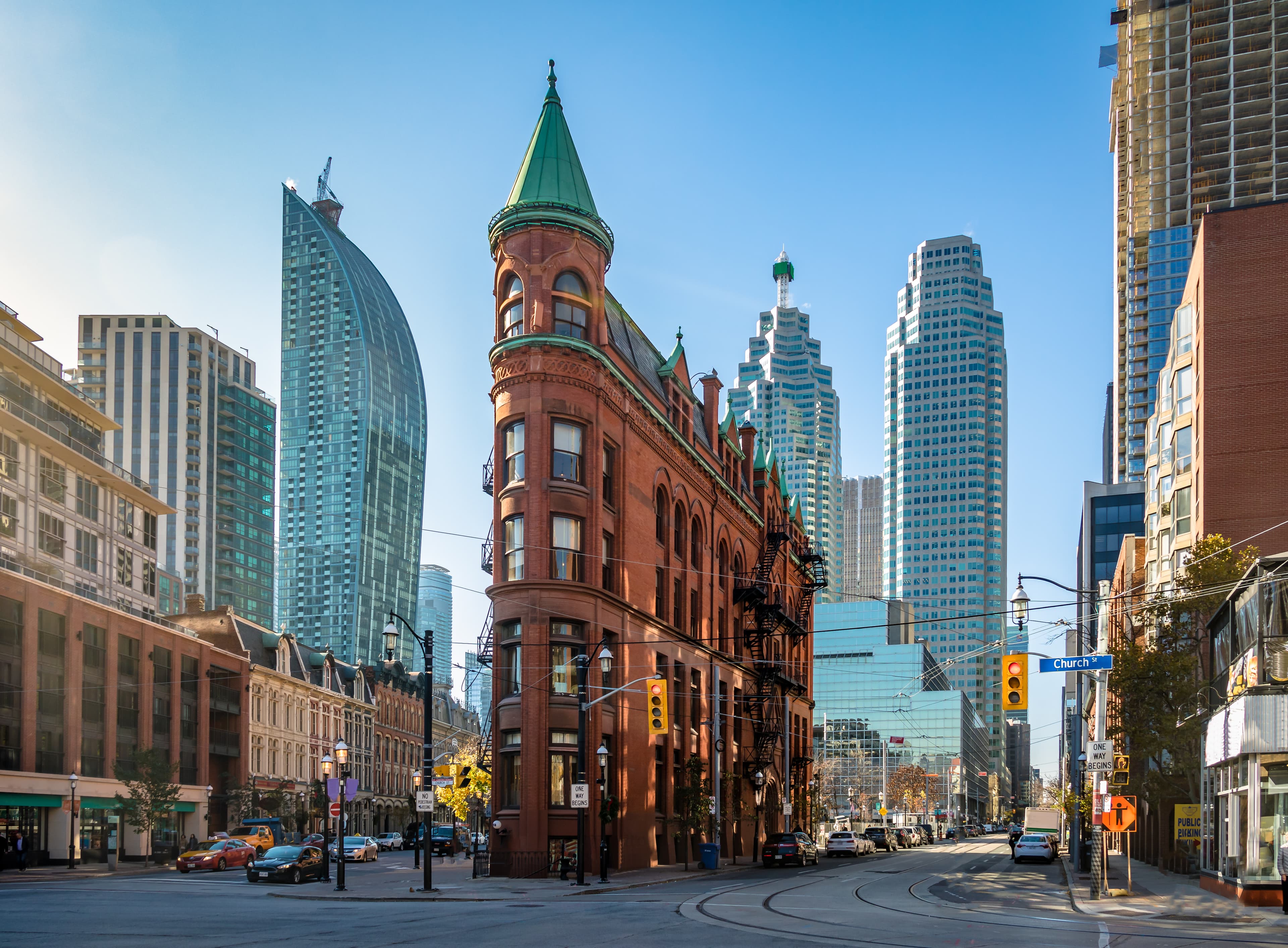
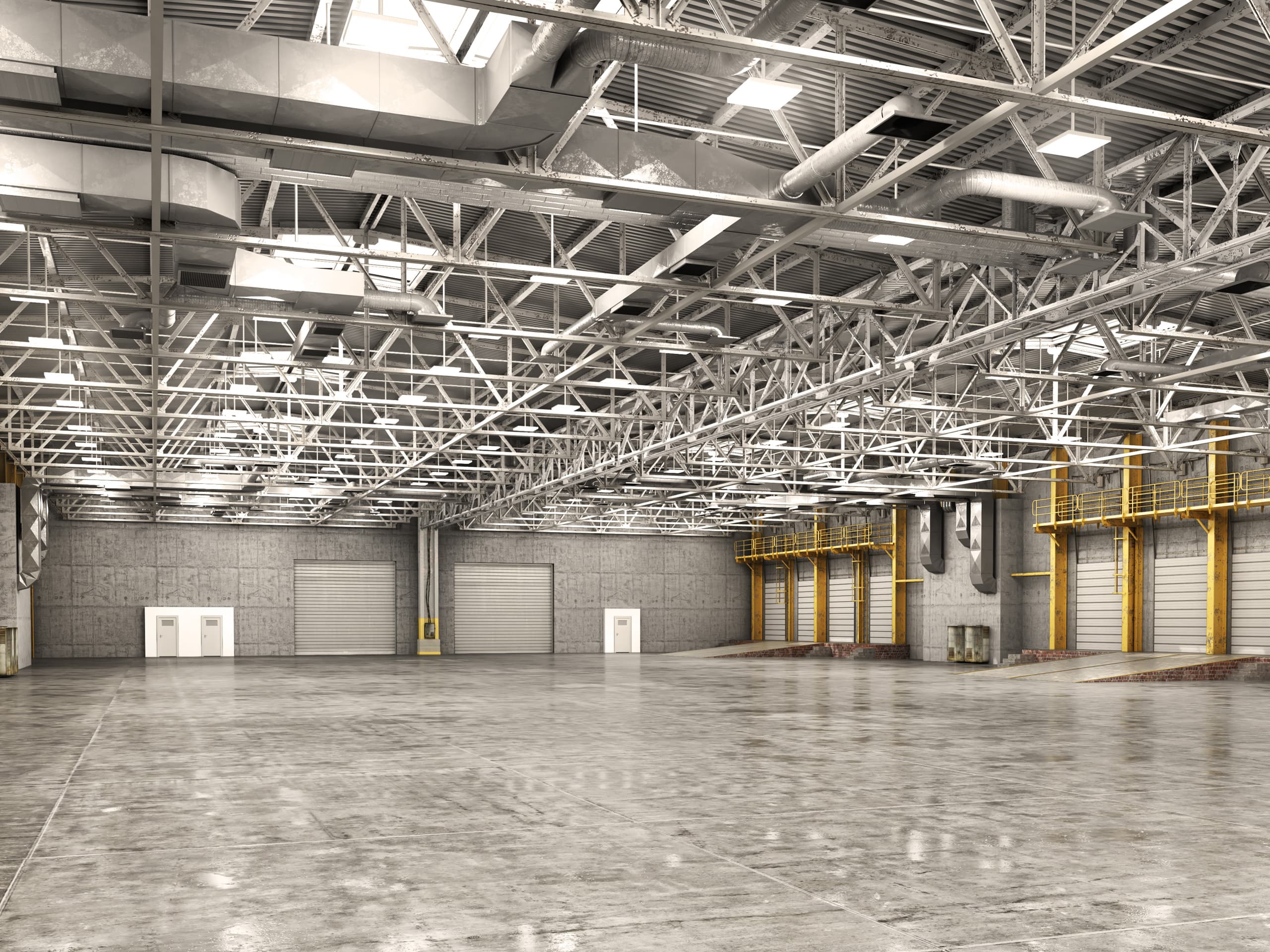
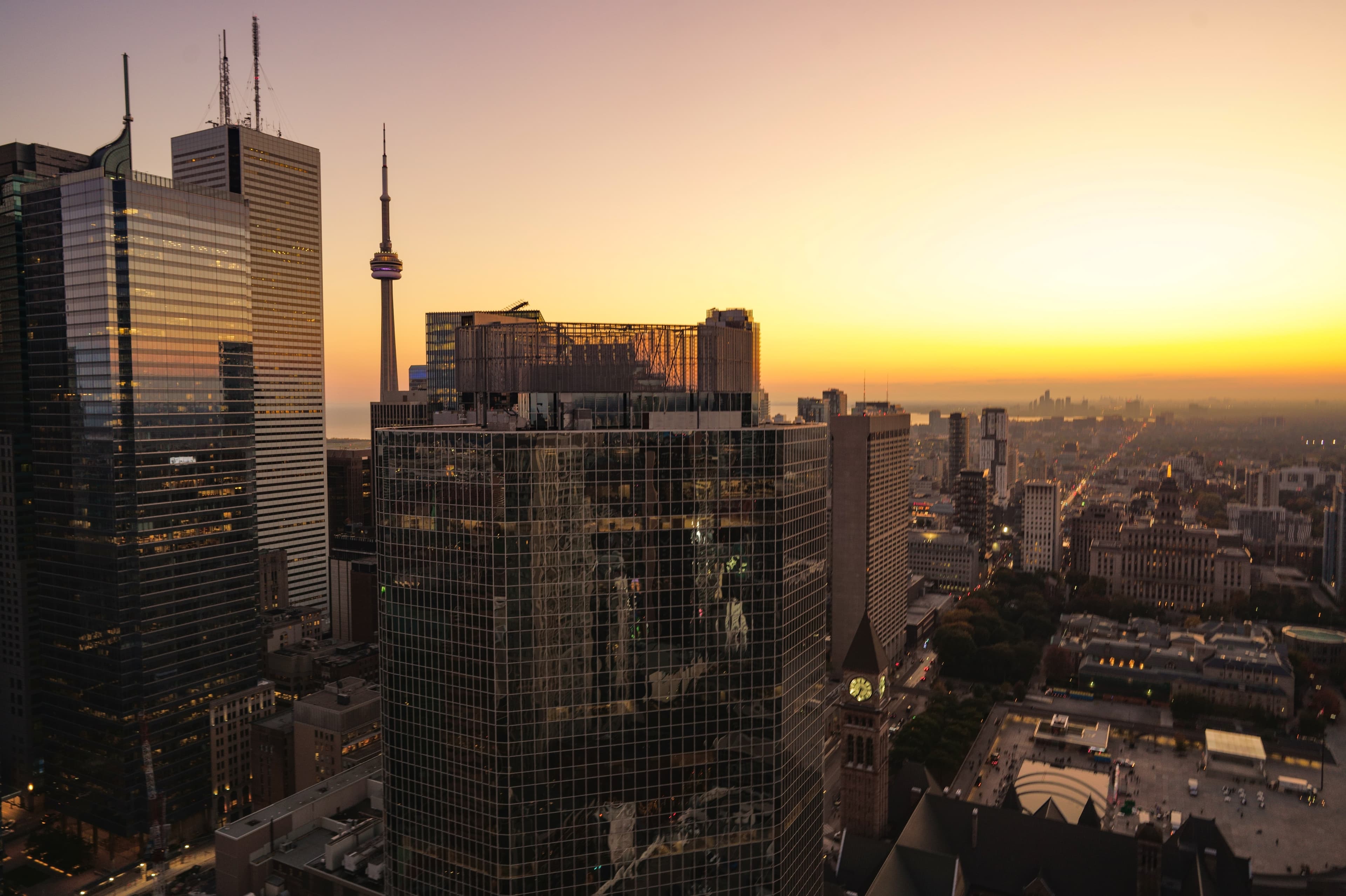