The surprising relationship between health insurance and the performance of your CRE properties
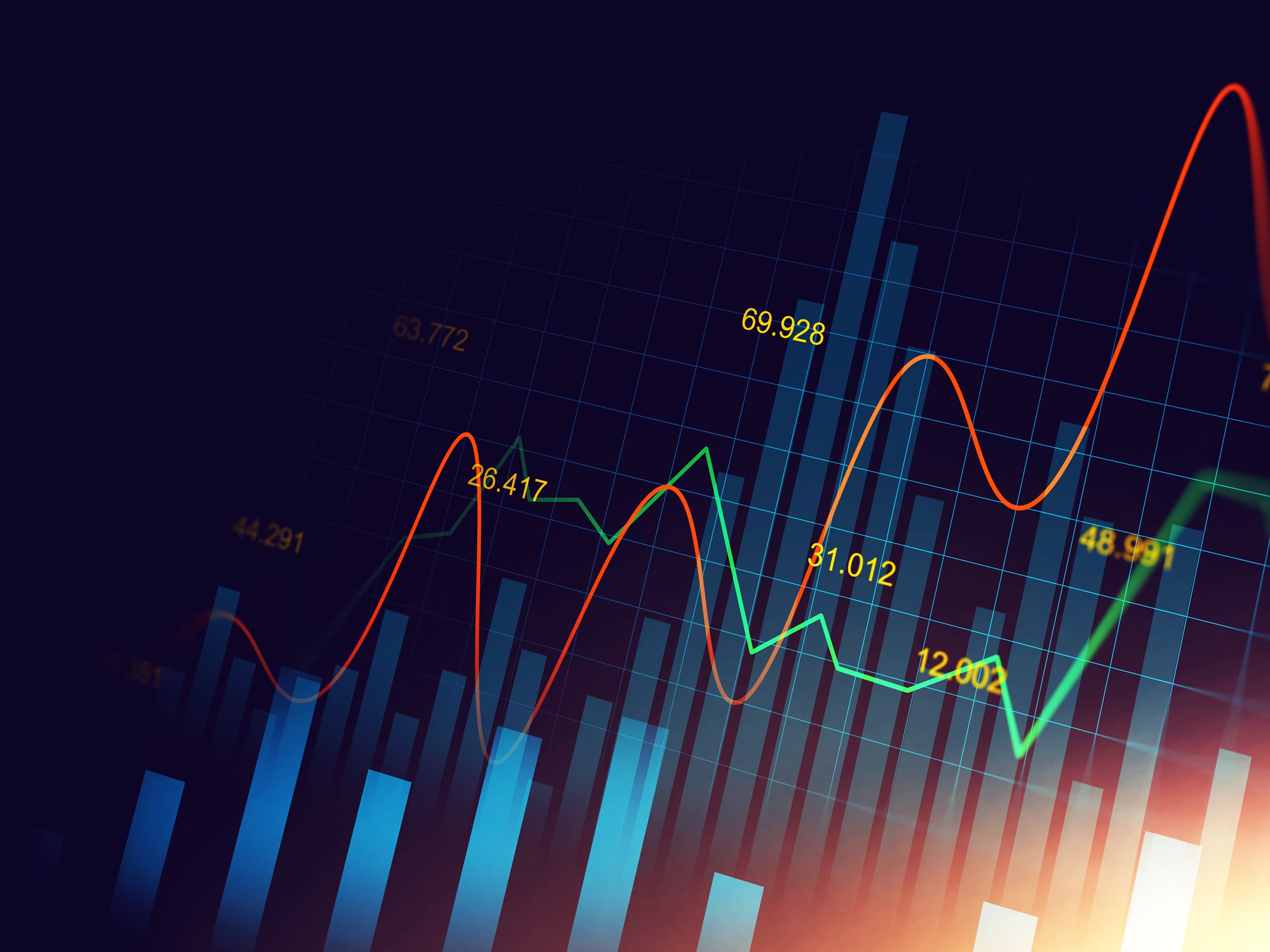
Commercial real estate firms are well-practiced in assessing the current state of markets. But predicting future returns? Relying on instinct and retrospective data has proved to be challenging.
For example, what factors should you examine to determine an asset’s performance vulnerability in the event of an economic downturn?
Macroeconomic and demographic metrics such as unemployment rate, population growth, regional GDP, median household income, growth in median home value are often lagged indicators – making them limited in their ability to yield timely insights about an asset’s net operating income (NOI) in a potential recession.
But using a machine learning platform where data scientists can combine traditional valuation and performance data with more granular market and economic data has started to identify new patterns and correlations between the data sets. One particularly striking example is the correlation between local population without health insurance and potentially weaker property-level performance.
Looking at the population near a property, the percentage of people without health insurance coverage is a stronger predictor of asset performance vulnerability than more commonly consulted factors, like the unemployment rate and local market economic growth.
Health insurance? Why is this a predictor of asset performance?
Consider that health insurance has a strong relationship with the unemployment rate. In the US, a majority of the population relies on an employer-provided system for a high proportion of insurance coverage. The unemployment rate used most widely, the U-3 unemployment rate from the U.S. Bureau of Labor Statistics, measures the number of people who are jobless and actively seeking employment.
Interestingly, the machine learning models were able to identify that the percentage of the uninsured population proves to be a stronger indicator of employment fragility or strength within a region or neighborhood compared to the U-3 unemployment rate.
Why? Because an individual who cobbles together two part-time jobs or earns their living as a gig-economy worker won’t show up in the U-3 unemployment rate. But they’re more likely to show up in a measure of the uninsured population.
So, it turns out that a higher level of employment instability near a multifamily real estate property is a better predictor of future (under)performance than the use of the unemployment rate.
Of course, part of this relationship to property performance is driven by the compression of the unemployment rate in the recent white-hot labor market. Still, as we face a potential macroeconomic downturn, the rate of the uninsured population around a property is likely to serve as a predictor of near-term asset performance vulnerability for multifamily properties.
Proving the point: testing the rate of the uninsured population, and other factors, against property-level performance
It’s enlightening to see how the validity and reliability of this relationship is determined. The concept of interpretable machine learning becomes critical in a circumstance like this, where the initial finding and impact on models doesn't initially line up with human models of the past.
Below is a brief overview of some of the mathematical mechanics that led the machine to pull out healthcare insurance coverage as a factor related to commercial real estate returns.
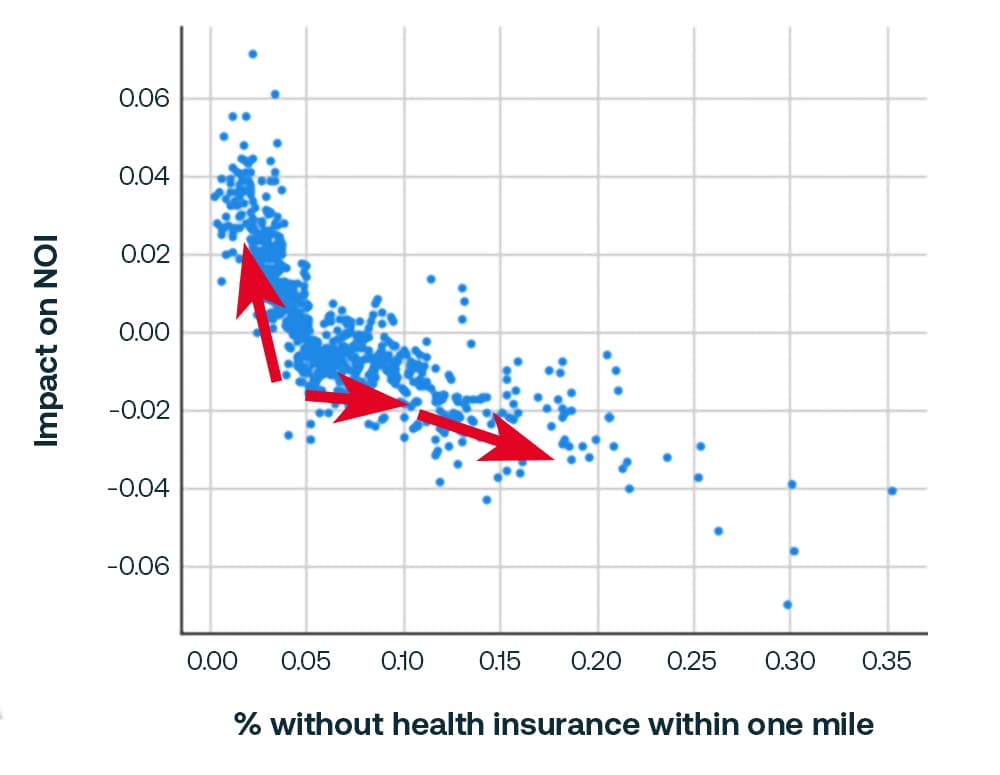
The percentage of uninsured local population impacts NOI for multifamily property.
The scatterplot above depicts a group of multifamily properties. The mathematical relationship between the NOI of these properties and their surrounding population without health insurance tend to fall in line with the following patterns.
<5% uninsured: Compared to similar types of properties, when less than 5% of the population within a one-mile radius around a property has no health insurance, it tends to experience a lift in NOI.
5-10% uninsured: When the percentage of uninsured residents rises above 5%, there tends to be a pronounced negative impact on NOI: -1%. This -1% performance drag remains steady when 5%-10% of the surrounding population is uninsured.
>10% uninsured: As the rate of uninsured rises above 10%, there tends to be an increasingly negative downward trajectory of NOI.
Measuring how the uninsured factor impacts NOI in context with other factors
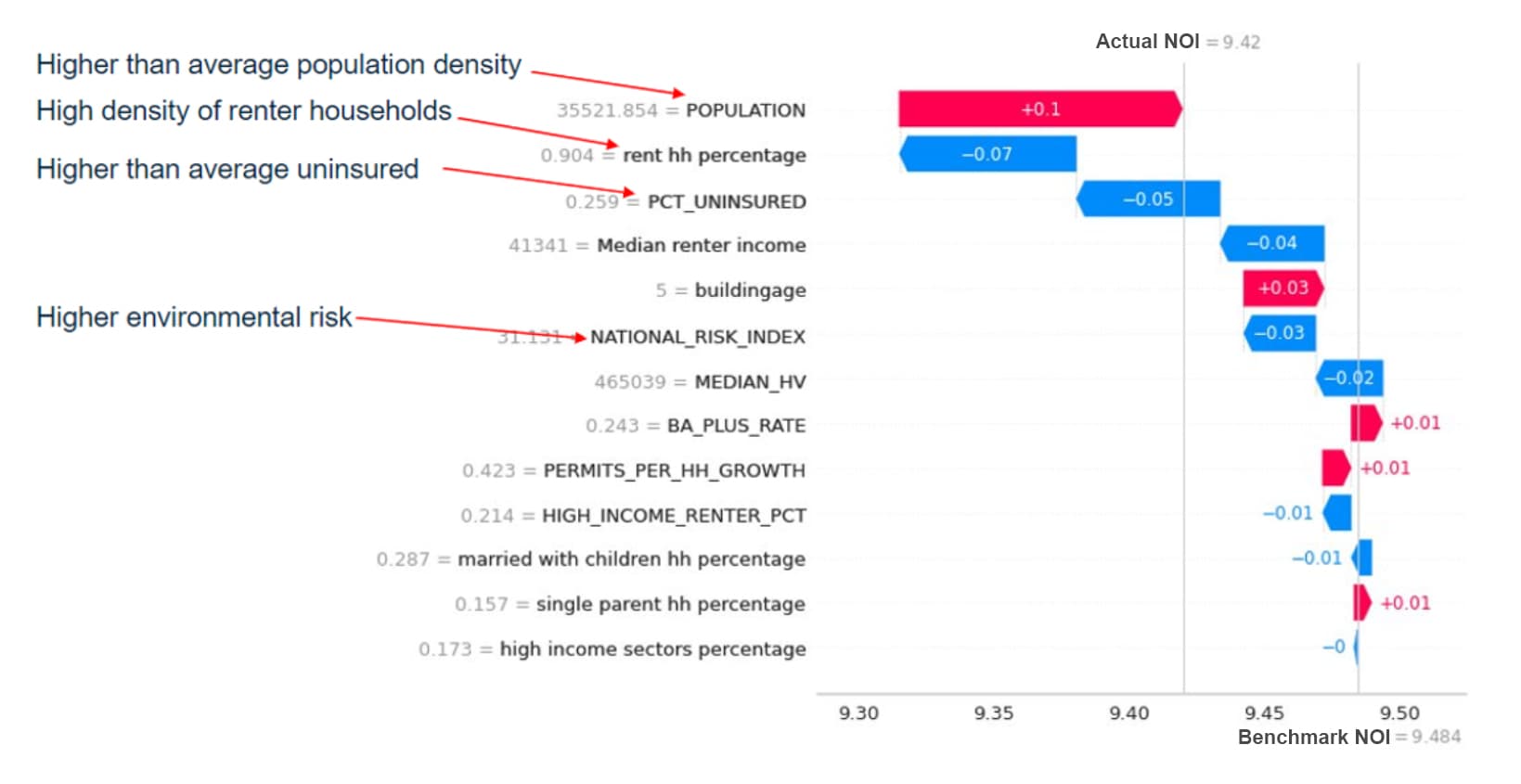
The example above explained how asset performance could be modelled using the health insurance coverage ratios, but this is only one example of a factor which has been correlated with NOI growth. Introducing more data into the model allows us to build additional models and advances how we view commercial real estate performance because we can see how these factors impact NOI and how they interact in context with other factors.
Through machine learning, we can scan dozens or hundreds of hyper-localized macroeconomic and demographic factors and property-level performance factors to determine which of those factors have any impact on NOI for a specific property.
Then for the factors that impact NOI, the machine learning platform can perform Shapley Value analysis that isolates the relative performance gains and costs that those factors impose on NOI, in context of the impacts that other factors have on that property.
The waterfall chart below, for example, shows the combined results of 13 factors that sometimes impact performance for multifamily properties, identifying the relative contribution that each of those factors have on the NOI performance for a specific multifamily property example.
This chart shows how several market factors, which are traditionally used for property analysis, can in fact sharpen models for NOI performance for this property:
Ratio of permits vs. future population growth
Population with college degrees
Median home value
Building age
Median renter income
The latter is the most significant of these traditional factors, impacting NOI performance of this multifamily property by -4%.
Yet three other factors demonstrate even greater impact.
Population density
The macro factor with the strongest impact on this property is population density. When compared to similar types of properties, the neighborhood in which the property was located had higher than average population density which was expected to result in a 10% higher NOI. However, this was only one market factor which was predicted to impact NOI performance and the other two – density of renter households and uninsured population negatively impact NOI growth.
Density of renter households
The impact of renter density is not a linear relationship – it only matters in certain circumstances, such as when density crosses a specific threshold, at which point the market sharply penalizes returns when an extremely high density of renters around this specific property reduces performance by a substantial 7%. And so, this factor doesn’t typically have a material impact for most properties because they aren’t in neighborhoods that are that dense with renter households.
Uninsured population
The third significant factor deflating performance is the rate of uninsured population surrounding the property, impacting it by 5%. In combination, the density of renter households and the percentage of uninsured population mitigate the predicted gains from being located in a high population density location.
How CRE firms can leverage this economic factor today
How can you best employ data like health insurance rates to predict returns for your commercial real estate properties?
The availability of this data and the ability to analyze it on a machine-learning platform can yield much more rapid analysis of highly complex relationships than what is possible with manual analysis. And in the CRE industry, being able to identify a unique or hidden market pattern and becoming a first mover on an under-the-radar opportunity can yield considerable advantage.
Properties in neighborhoods with a higher-than-normal rate of uninsured population may suffer from the greater level of employment instability in a downturn. So these might be good candidates for divestment while the market is still priced higher. Alternatively, taking proactive steps today to ensure your asset and property managers are monitoring tenants and market risks to manage this risk can avoid future challenges in the event of an economic downturn.
For example, for fund and portfolio managers who are concerned about recession risk, understanding which assets may be exposed to risk factors, such as the percentage of the uninsured population, can help inform a strategy around your current properties or potential acquisitions.
New ways to optimize real estate performance, uncover risks and opportunities
Including health insurance as a factor to sharpen predictions for performance vulnerability is merely scratching the surface, considering what advancements in data science are revealing between market factors and asset performance.
Overall, when it comes to more precisely identifying the factors impacting the performance of your commercial real estate assets, data science can play an invaluable role in validating, or invalidating, your hypotheses and intuition-based models.
CRE firms can now conduct in-depth analyses to determine, among an extensive range of factors, which ones most accurately predict asset returns. Moreover, as well as identifying those factors that materially impact a specific property, it is also possible to quantify their expected impact and relative importance.
By harnessing data science – traditional and alternative data sets, machine learning technologies and predictive analytics – CRE firms will be well positioned to time investments and divestments for optimal returns, to enhance property performance and to uncover hidden risks and opportunities.
Author
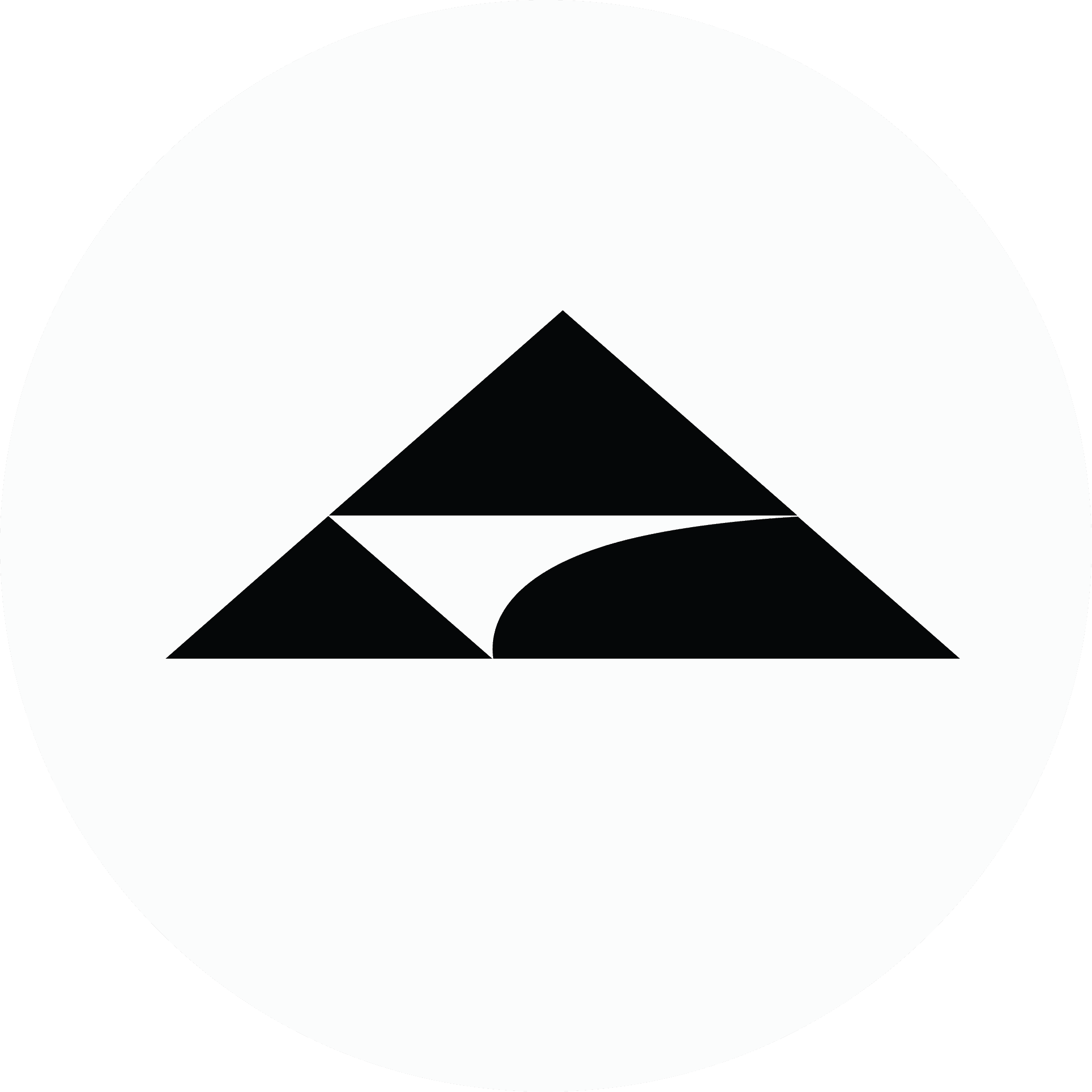
Altus Group
Author
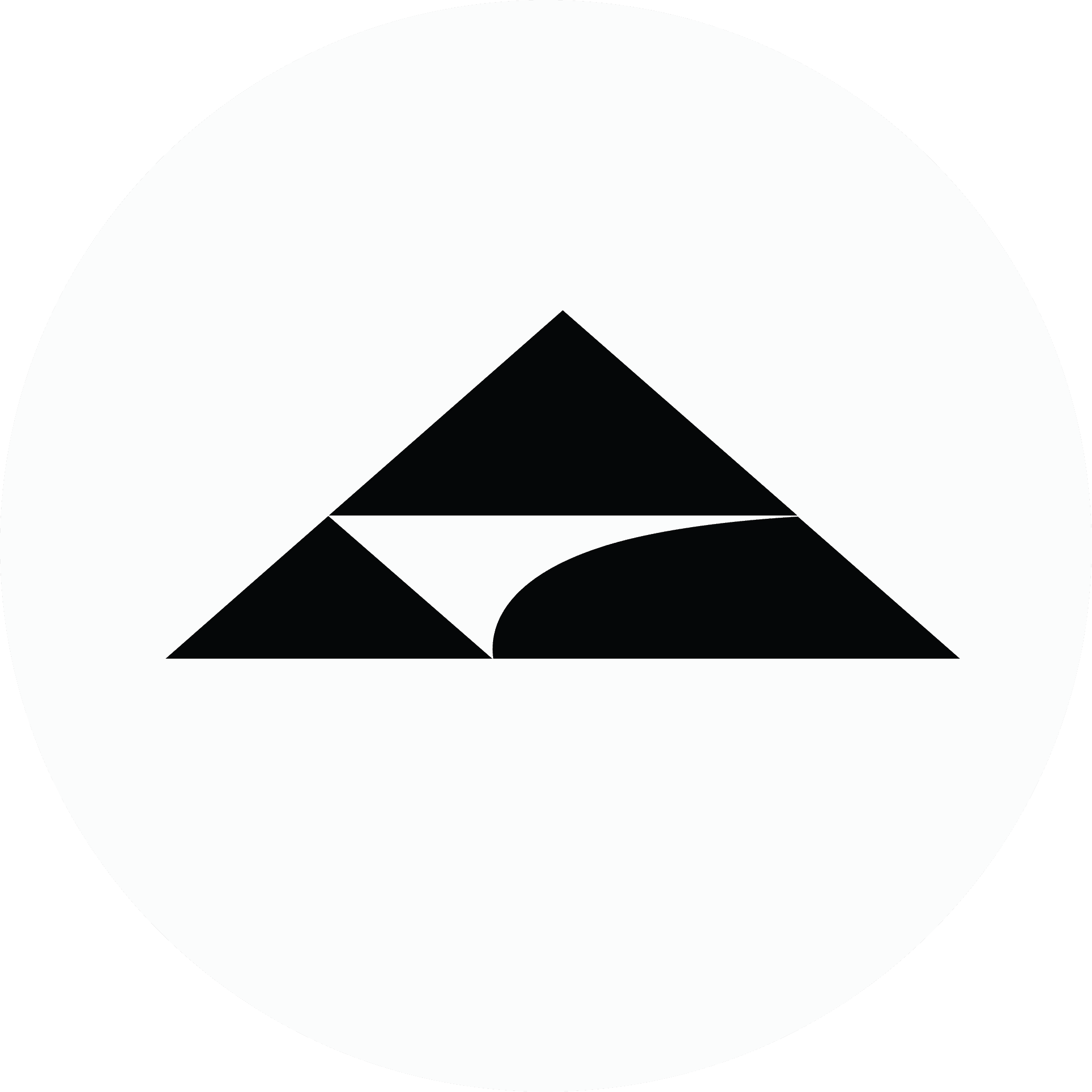
Altus Group
Resources
Latest insights
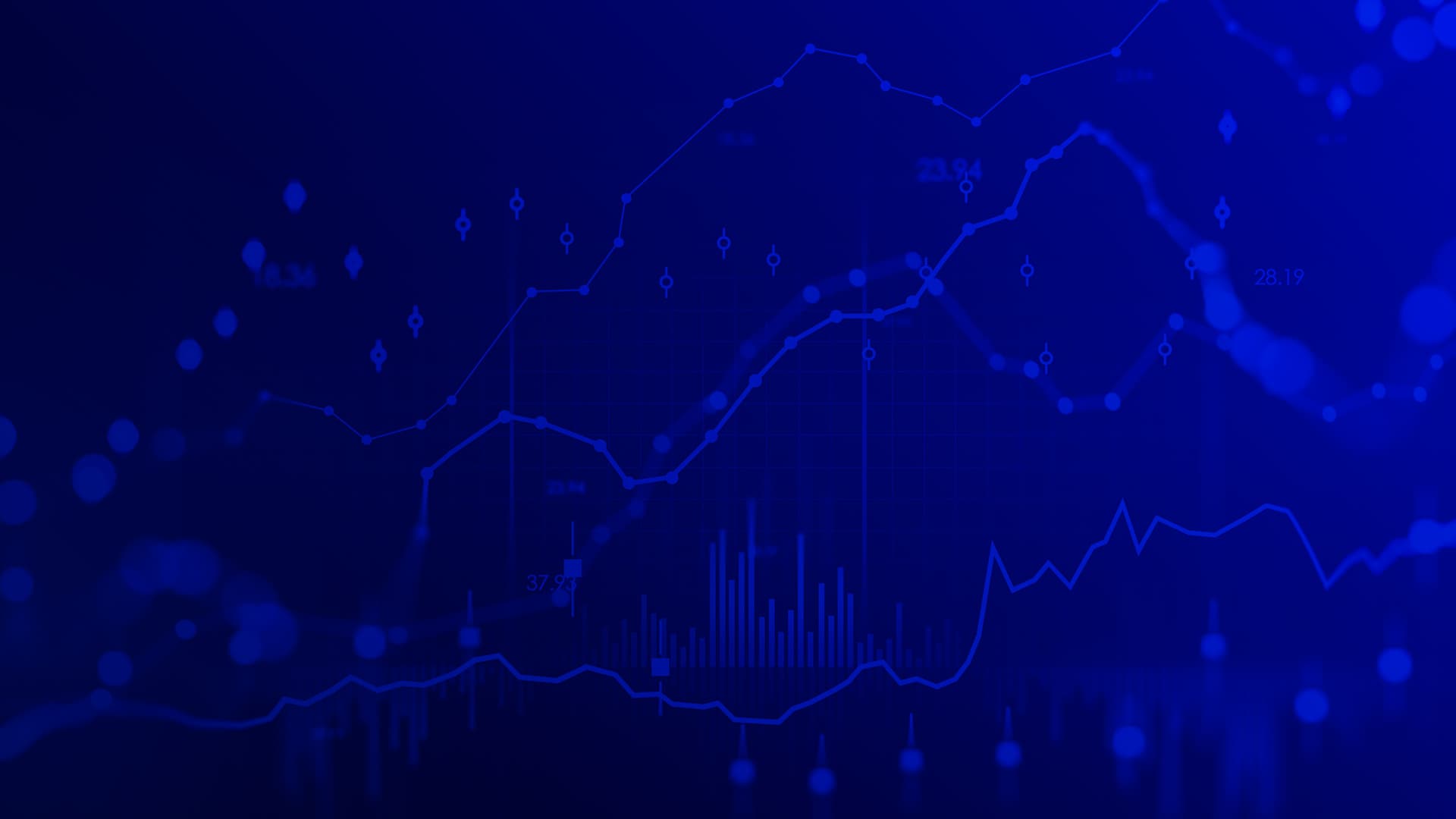
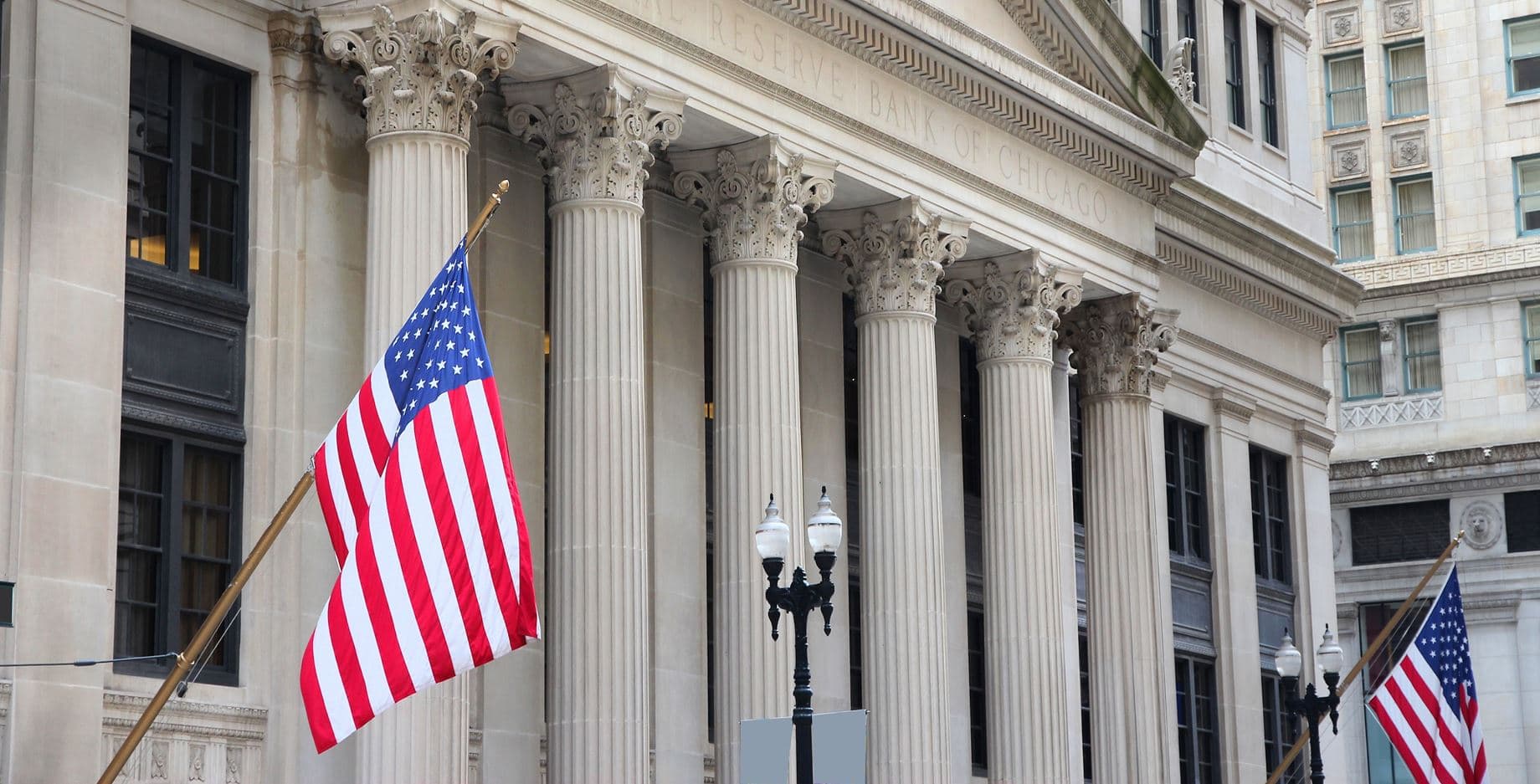
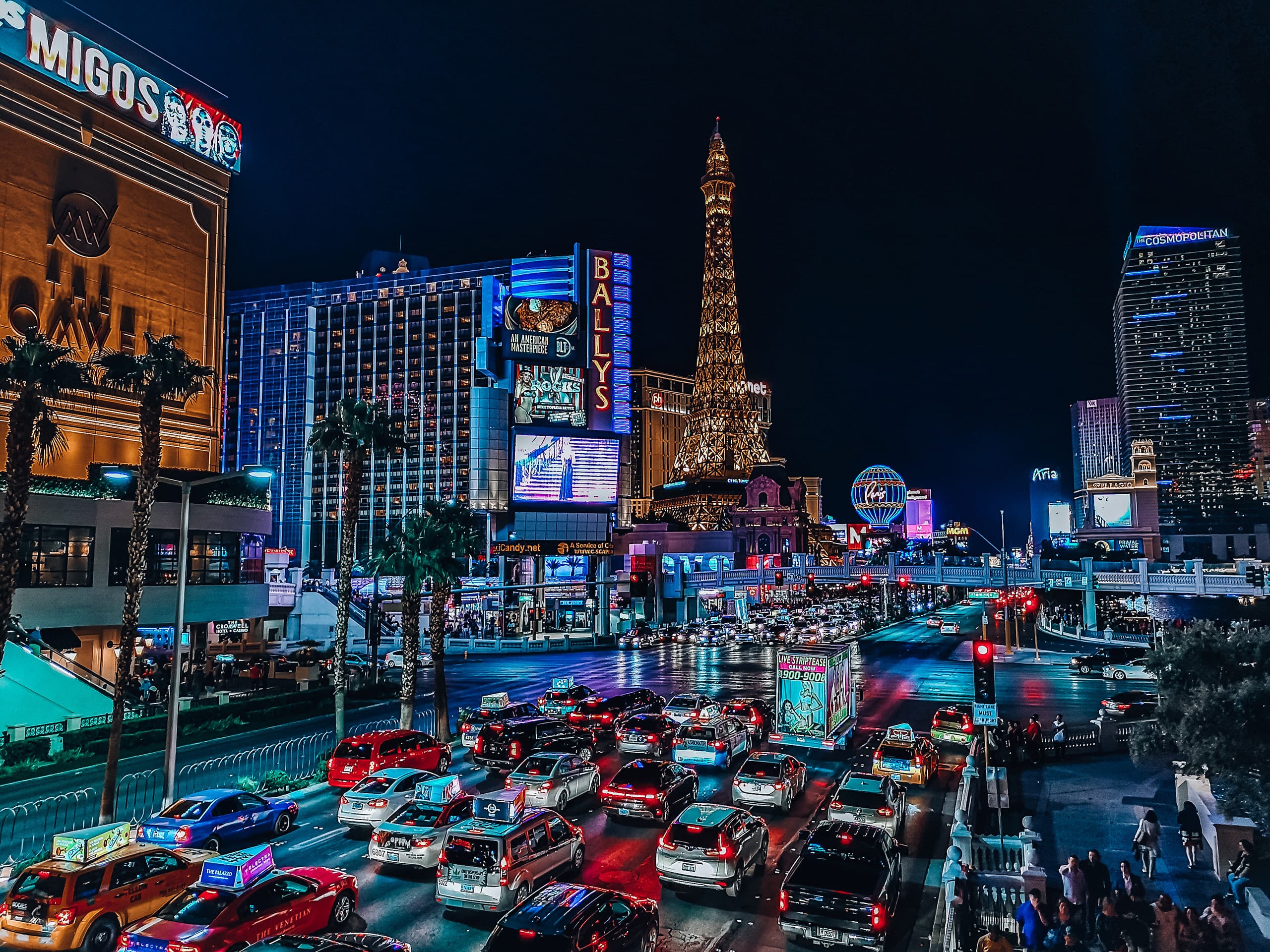
Nov 28, 2024