Key highlights
More than half (55%) of commercial real estate investors surveyed by Altus Group say they employ data science and advanced data analytics tools and techniques to support their investment decisions.
But Altus Group’s global research report, The state of data science in commercial real estate investing, also reveals a distorted perception of data science and advanced data analytics adoption.
66% of respondents across Asia Pacific, 78% in Australia and 73% globally describe their firms as being “ahead of the curve” of data science adoption.
So, what’s the real data science story in commercial real estate? Altus Group asked Investa’s General Manager for Innovation & Advanced Analytics Joanna Marsh for her insights.
When it comes to commercial real estate data analytics and data science, 34% of global commercial real estate companies think of themselves as “innovators and risk takers”.
But Altus Group’s survey – which captured the views of 400-plus from commercial real estate investment companies in 14 countries, 25% of those in Asia Pacific – finds no correlation between perception and reality.
In fact, many respondents who viewed their companies as innovators or pioneers in data science and analytics were less likely than their peers to have developed advanced data science capabilities.
Figure 1 - Organization’s approach and attitude towards data science/analytics tools and technologies for real estate investments
Figure 2 - Percentage of firms at each perceived level of sophistication using analytics capabilities only versus use genuine data science capabilities
This doesn’t surprise Investa’s General Manager for Innovation & Advanced Analytics, Joanna Marsh. She has spent the last two decades in real estate, the last three years of those developing sophisticated commercial real estate data analytics and machine learning models to aid research, capital allocation, asset acquisition and leasing. Joanna thinks real estate is where the financial sector was in the 1960s.
“There is no Bloomberg for real estate,” Joanna notes. Real estate is “non-fungible and idiosyncratic” and most assets are privately held. Data is often not publicly available or easy to aggregate, even in transparent markets, which means real estate decision-making is still “highly reliant on human intel and bits of information”.
But all asset classes are being transformed by data science, and Joanna points to professional sports teams that now scout with applied machine learning as an instructive example. “There’s nothing more non-fungible than a human. If you can use data science for a human soccer player, you can for real estate.”
Building the best commercial real estate data foundations
Altus Group’s survey shows that half of all commercial real estate investment firms have established some form of internal data science or analytics capability. Another 21% are planning to invest in developing these capabilities over the next two years. Among the 50% with established internal resources, the most common in-house capabilities involved the development of metrics, scorecards, new tools and technologies.
Figure 3 - Data science and analytics capabilities organizations currently employ to support real estate decision-making
But many companies conflate data science with data analytics. “Even companies with sizable data science teams are often spending most of their time on data engineering – they are getting sucked into reporting – rather than data science,” Joanna notes. Dabbling with data science is not the same as solving the “fundamentals,” Joanna notes. Laying the foundation for data science can take years of data collection and cleaning, optimising data storage, trialling and tweaking algorithms, and more.
Without these foundations, many companies are asking the wrong questions, in some cases, “getting really wrong answers”. Online real estate marketplace Zillow, for instance, wrote down US$304 million in late 2021 and exited the home-flipping business after unintentionally purchasing homes at inflated prices, based on machine learning algorithms.
“You can write a model that will give you indicators and help with decision support. But you can’t write a model that will tell you ‘this building is worth X’, because it will have a different value to different investors.”
Making smarter “more mechanical decisions” is easier at the operational phase, Joanna observes. Investa’s 60 Martin Place in Sydney, for instance, is bristling with more than 22,000 sensors that support building operations and preventative maintenance. But “up to 70% of total investment return is realised early in the real estate cycle – during market selection and capital allocation. “Sensor and building operational data are important. They form a key part of a good data intelligence strategy that allows you to respond to customers and iterative your design. But you can’t claw value back with sensor data.”
Hunting in the woods of commercial real estate data
Investa has teamed up with Oxford Properties to build what Joanna calls “hunting machines”. These are essentially sophisticated algorithms that can be “pointed” at any real estate market and can act as “listening posts” for a particular investment thesis.
The hunting machines analyse decades of commercial real estate data – from rents, leases and valuations to demographic information, like school profiles, geographic insights and development application histories – and can spot imperceptible market movements to give Investa and Oxford an investment advantage.
“In Sydney there are 50,000 assets across commercial office, industrial and retail and we listen to all of them,” Joanna says. Taking on this otherwise impossible task allows human talent to do what they do best – connect, collaborate, negotiate and seal deals.
Altus Group’s report found the top reason for investing in data science and analytics was to “gain competitive advantages and differentiate from our competitors”. But competitive advantage, Joanna suggests, isn’t found in the data points themselves, but in the human analysis.
Joanna was recently appointed CEO of Exomnia, a data analytics start-up she co-founded while continuing to maintain her day-to-day role at Investa in a part time capacity. It’s a concept Joanna calls “having a portfolio career”. Exomnia is developing a modularised approach to data analytics and allows people to create, customise, or simply ‘plug and play’ with a range of custom-built analytics packages. “Then human analysts don’t have to pull all-nighters plugging data into Excel spreadsheets. We can democratise data and all start asking really good questions.” This is especially important in the context of environmental, social and governance (ESG) value, Joanna notes.
Connecting commercial real estate data science with strategy
Altus Group’s survey suggests that large firms – especially those with US$1 billion or more in assets under management – are those setting the pace with internal data science capabilities. Large firms are significantly more likely to have internal data science teams in place, to have appointed chief data officers and to have developed internal, proprietary predictive models.
Figure 4 - Metrics from selected survey questions indicating that large firms are likely leading the way in internal development of data science
Figure 5 - Share of organizations with dedicated internal data science teams and proprietary predictive models by region
But Joanna offers a word of warning. “Just because you have a data science capability, doesn’t mean it is being adopted throughout your company. There’s often a disconnect. Capital transaction and leasing teams have spent their careers immersed in their local markets and asset classes, and they have a deep understanding of the drivers of value. It is vital to make sure that your teams are talking to each other; that the tech teams are building useful tech and that the business teams are actually using it.
“People in real estate have also been burnt after buying black box AI from other industries that tells them something they already know – like buildings with larger lobbies get higher rents. And the only way you can afford to do a big lobby is because your building can support higher rents, so it’s a self-licking ice cream. And if that’s all that comes out after spending two years and $2 million, the CEO says ‘cut the program’.”
It’s unsurprising, then, that Altus Group’s research reveals “unrealistic assumptions or expectations” as the biggest barrier to rapid deployment of commercial real estate data science, especially in Asia Pacific.
Data science is a long-term investment, Joanna observes, and it can be difficult to demonstrate ROI. “Data science is expensive and it takes a long time to get a result. If you trade an office asset every seven years, then it’s seven years before you get the proof point.”
What’s the solution? “Start small,” Joanna says. Look for the quick wins and then iterate. Investa has a prioritisation framework so data science projects solve obvious business pain points and are aligned with corporate strategy. “That’s how you get sponsorship and support.”
Author
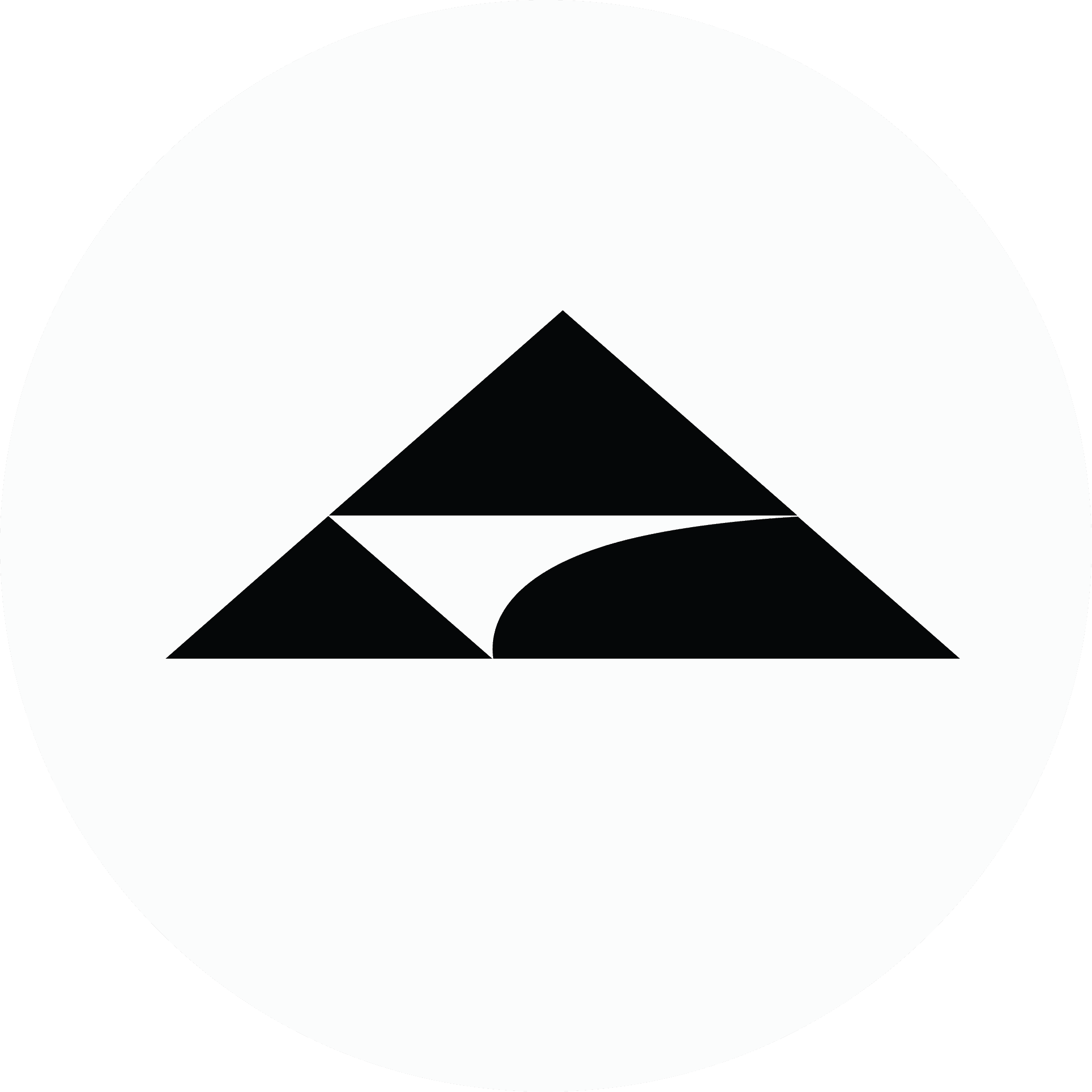
Altus Group
Author
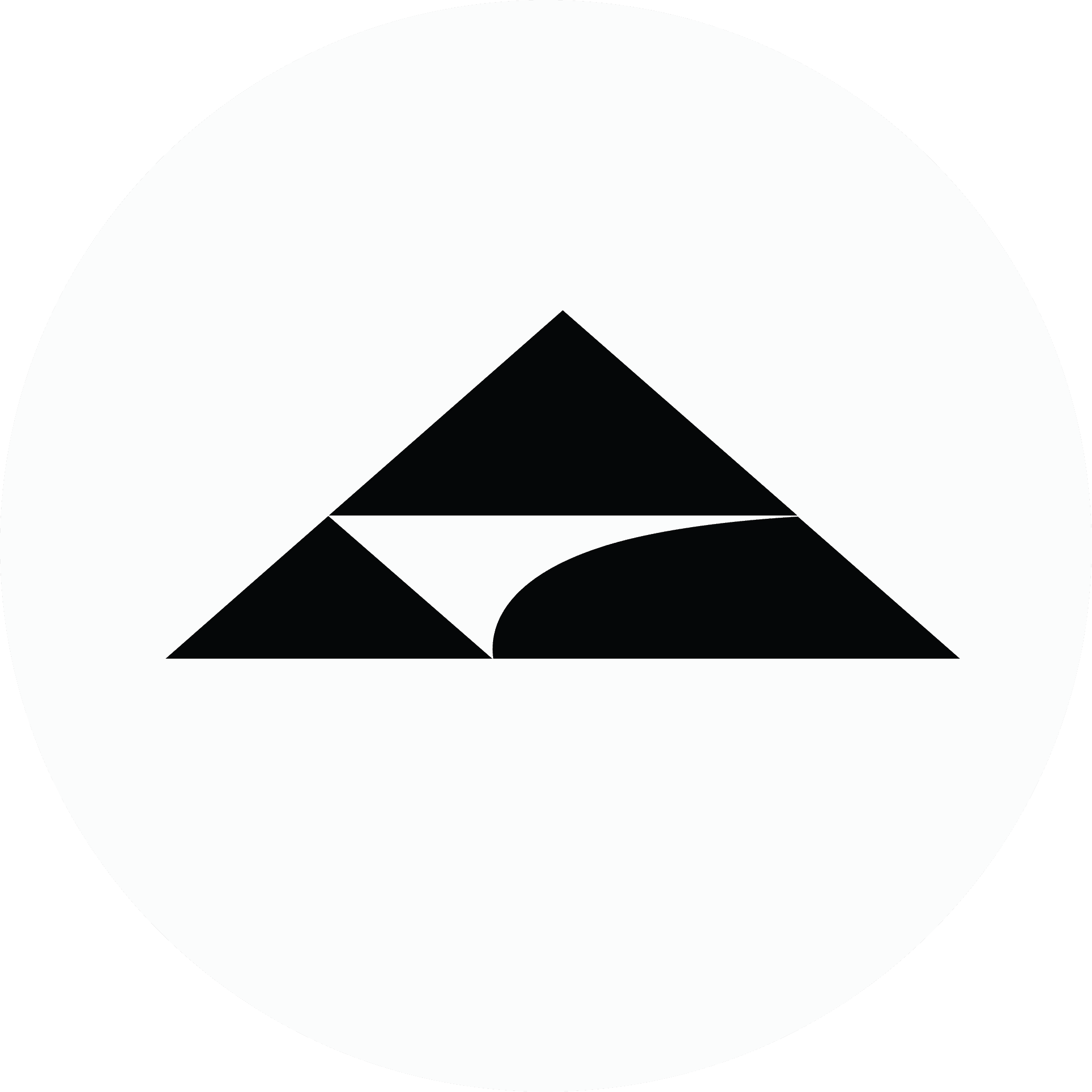
Altus Group
Resources
Latest insights
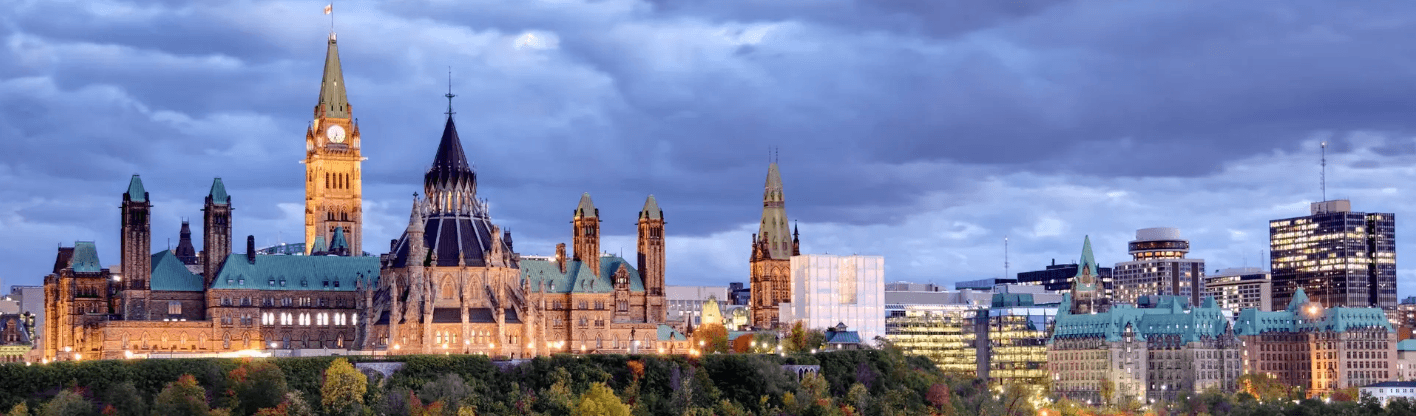
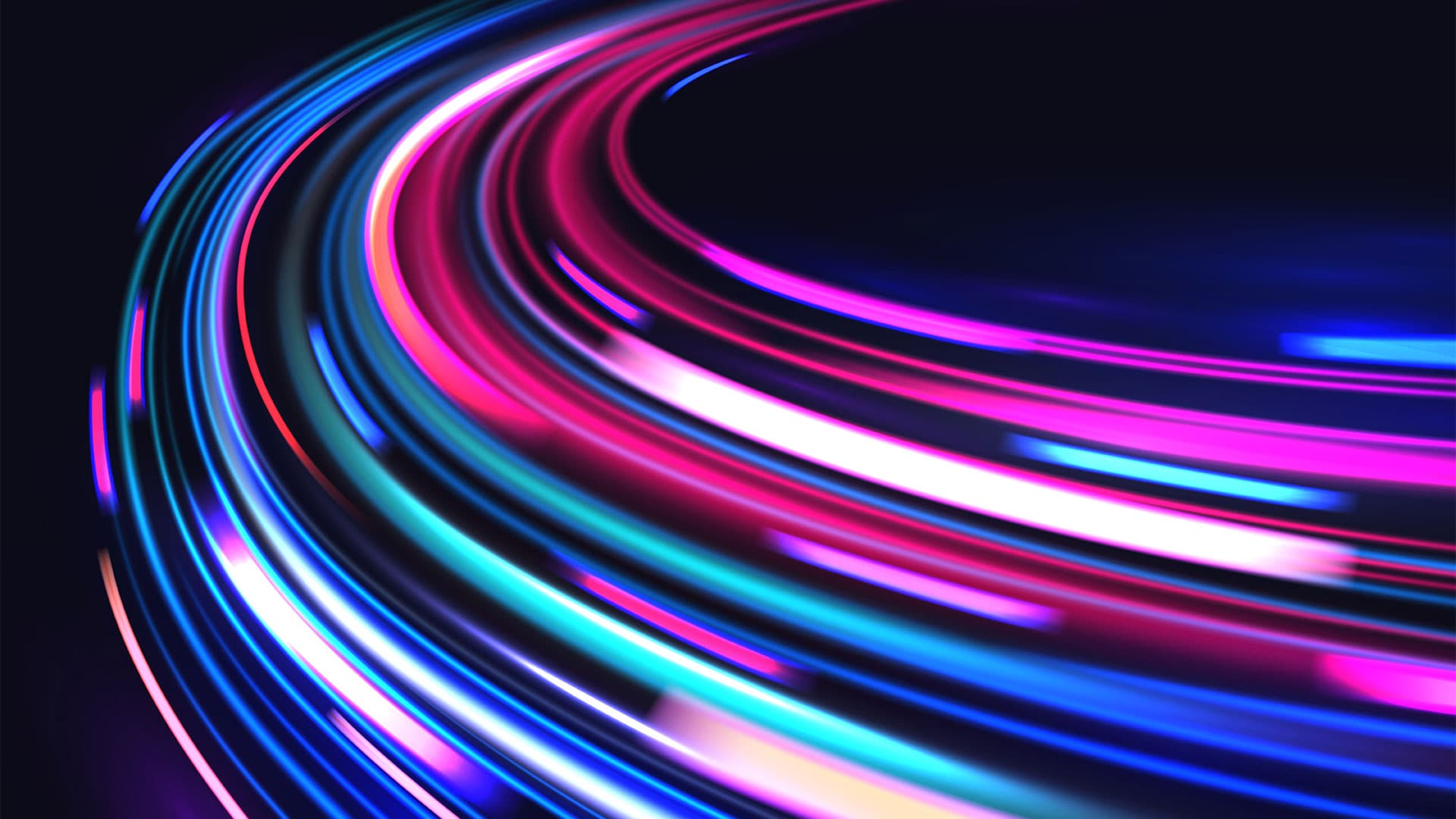
Mar 20, 2025
Innovation in CRE benchmarking is ushering in a new era of data-driven decision making
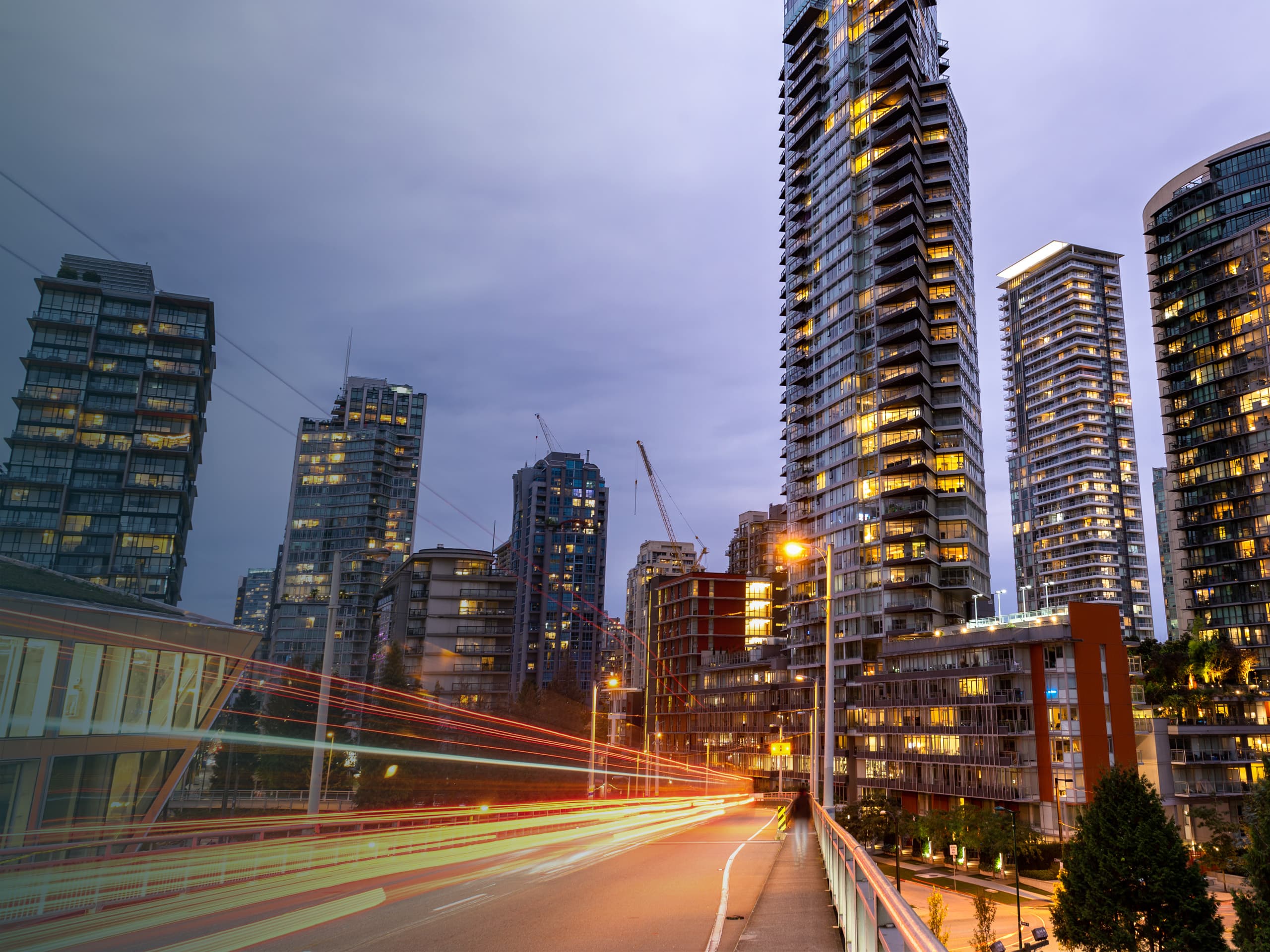
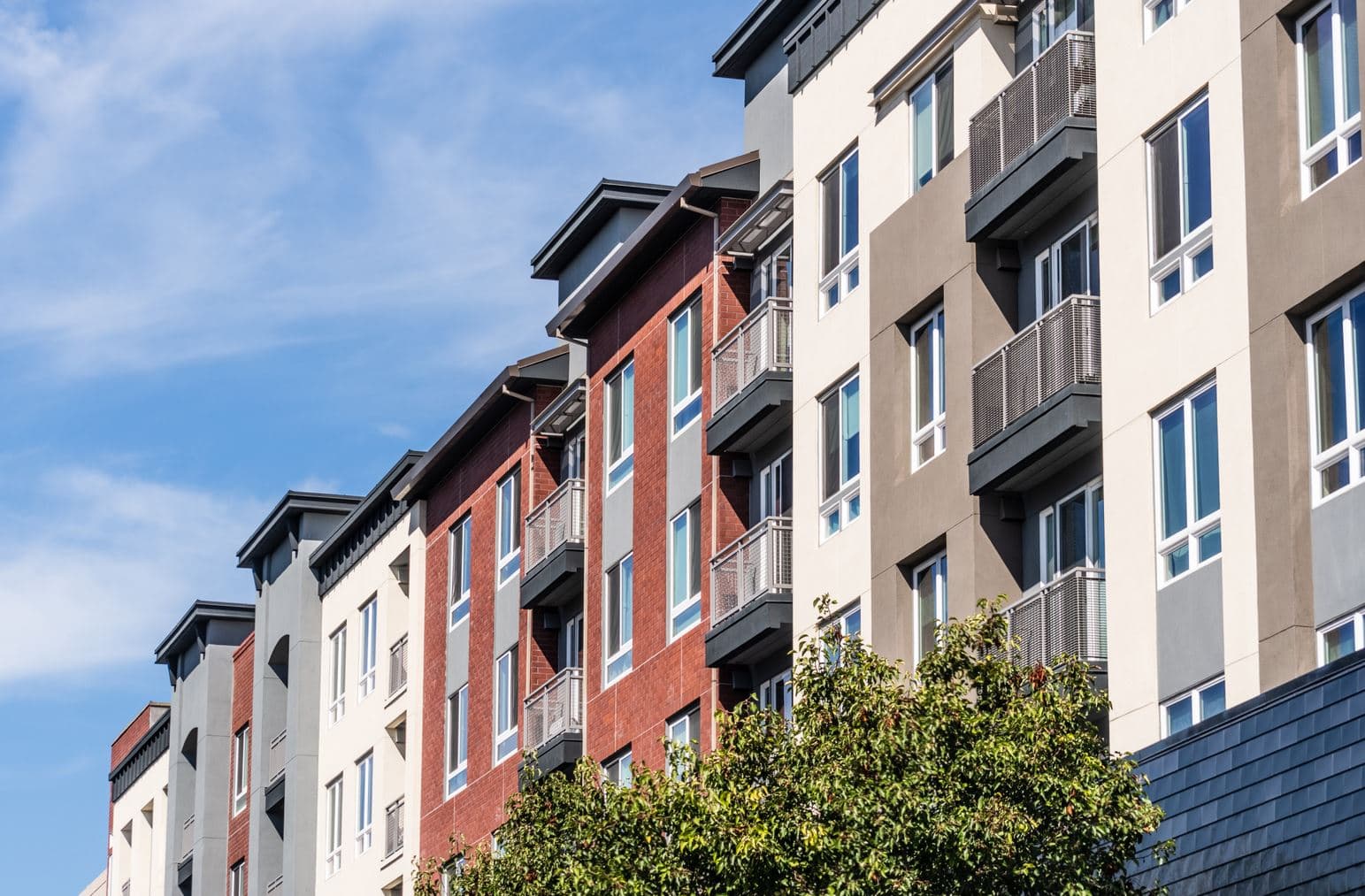